Although virtual interviews have been around for some time, the current pandemic has caused unprecedented demand for video-based meetings and interactions, including job interviews.
It is easy to see how this unexpected sequence of drastic events (i.e., a virus, a pandemic, social distancing, and, for those who are fortunate enough to keep their jobs, working from home) may lead to a long-term surge in adoption of digital recruitment tools, increasing the efficiency of interviews, and modernizing a rather outdated practice.
That said, it would be naïve to expect the widespread adoption of virtual interviews to translate into better recruitment practices. Faster, cheaper, more convenient job interviews won’t equate to more accurate hiring decisions, not unless human recruiters have the capacity to remove themselves from the process of judging and rating the actual interviews.
To be sure, some recruiters are exceptionally astute at making smart inferences of candidates’ talent and potential, and the same applies to a small percentage of hiring managers. But for each and every one of these intuitive mavericks with outstanding observational instincts, there are probably 100 who are much more inept and biased but think they are as good.
Make Job Interviews More Useful
In short, the main opportunity to make job interviews more useful is not to make adjustments to the actual interview process or candidate experience, but to debias the process of evaluating interviews. Since it is not possible to replicate the intuitive judgments of those who are just naturally good at reading people – you can’t clone an expert or reverse-engineer their experience – this is better done through technology, such as AI.
After all, the main advantage virtual interviews have over analogue interviews is that they generate vast amounts of data, so our main goal should be to translate those data into predictive insights.
Curiously, candidates, employers, and the general public seem reluctant to accept that an algorithm may be able to detect certain markers of potential (or signals of talent) from an interview performance. Yet that is exactly what human interviewers are trying to do when they examine interview candidates. Unfortunately, a great deal of evidence suggests that the average recruiter is not particularly good at this, to begin with.
First Impressions
First, we know that humans make very rapid inferences of others – after even a few minutes of interaction – and that we are rarely eager to change our initial impressions of others even when presented with clear evidence that we were wrong.
Second, most of the critical dimensions of talent – the attributes or competencies that make someone different from others and a great candidate for most jobs – are not directly observable, yet are the very things that we try to discern in interviews. For example, how can you objectively tell if someone has high levels of curiosity, integrity, or critical thinking? While recruiters are tasked with finding individuals that have these talents, there are many other tools available that can do this job far better than humans.
For example, psychometric diagnostics powered by AI can provide accurate, and bias-free, insights about one’s talent at a fraction of the time and cost of interviews (if you have 5 minutes, you can try one here).
Third, if there truly is a formula to connect what people say and do during an interview with their talent or potential (which, we know, can be done by correlating interview data to future performance indicators), then what makes us think that humans will be better at identifying those patterns than AI? An algorithm is just a formula, a recipe to identify patterns in data, and AI can do this at scale.
Humans, on the other hand, may successfully pick up one or two patterns (e.g., more eye contact = confidence or trustworthiness, fewer body movements = emotional stability, more questions = curiosity) and rather imperfectly. The main reason is not a lack of training or preparation for spotting key signals, but our total inability to ignore irrelevant signals (e.g., gender, age, race, and attractiveness).
AI Can Imitate Bias
In fact, for all the talk of AI being biased, it is not possible for a computer-generated algorithm to be biased in the way humans are. Computers don’t have fragile self-esteem they need to inflate by bringing other people (perhaps computers?) down. They are also not generalizing through false or biased categorizations, deductions, or inductions.
Computers don’t need unconscious bias training, for they have no unconscious bias. They also have no conscience – they just process data. Of course, their algorithms are only as accurate as the data they are fed, and we should be worried about monitoring this.
Garbage in, garbage out is an old adage in statistics to remind us that the quality of the data, as the raw ingredient needed for AI, is critical. This is why when AI has been trained to predict biased outcomes, it has not only imitated but also augmented human biases.
For example, you can easily train a machine-learning model to predict who is likely to get promoted in an organization. If the usual answer is “middle-aged white males” then it will be extremely effective at identifying whether someone fits into that category or not, and discriminate against anyone else.
A small detail that is often overlooked, however, is that such an algorithm would simply be exposing a pattern that already exists in the system. Clearly, we can complain about bias in the algorithm but if we stop using it then middle-aged white males still get promoted, as they were before we started using the algorithm.
It is therefore somewhat ironic that we are quick to accuse algorithms of biases that are just human biases. Instead of appreciating the fact that technology can be used to not just detect, but also combat, human biases. Surely we should be willing to accept an AI algorithm that we can test, monitor, and reduce its bias, over inherently biased humans who are resistant to change.
Easier Said than Done
This, of course, is easier said than done (which is true for everything). Without objective performance data – data that truly reflects employees’ contribution to the organization, and where they rank in the overall talent pool – it is very difficult to leverage the predictive power of AI. And in some instances, even seemingly objective markers of people’s performance may contain bias. A bias that exists in society rather than the organization.
For example, attractive people – and we can define attractive according to some relatively arbitrary cultural standards – will tend to outperform their peers. So should we hire individuals based on their looks?
By the same token, even though the world of talent is more meritocratic and less nepotistic than, say, 100 years ago, social class still predicts career advancement. Does this mean that we should train AI to select for, or ignore social class? These are complicated ethical questions that require a fairly good understanding of science and technology. The answer to these questions is not straightforward.
A good starting point is to have the humility and self-criticism to acknowledge the dominant double standards around virtual interviewing right now: we are hyper-critical and skeptical of AI, but appear to have very low standards when we judge the human part of the equation.
It is a bit like when one self-driving car crashes during a pilot exercise. We conclude very quickly that we must stop entertaining the idea of driverless cars. Yet at the same time, millions of people die every year courtesy of human-induced car fatalities, and that’s ok.
There is no question that AI is still work in progress. Humanity, on the other hand…
Authors
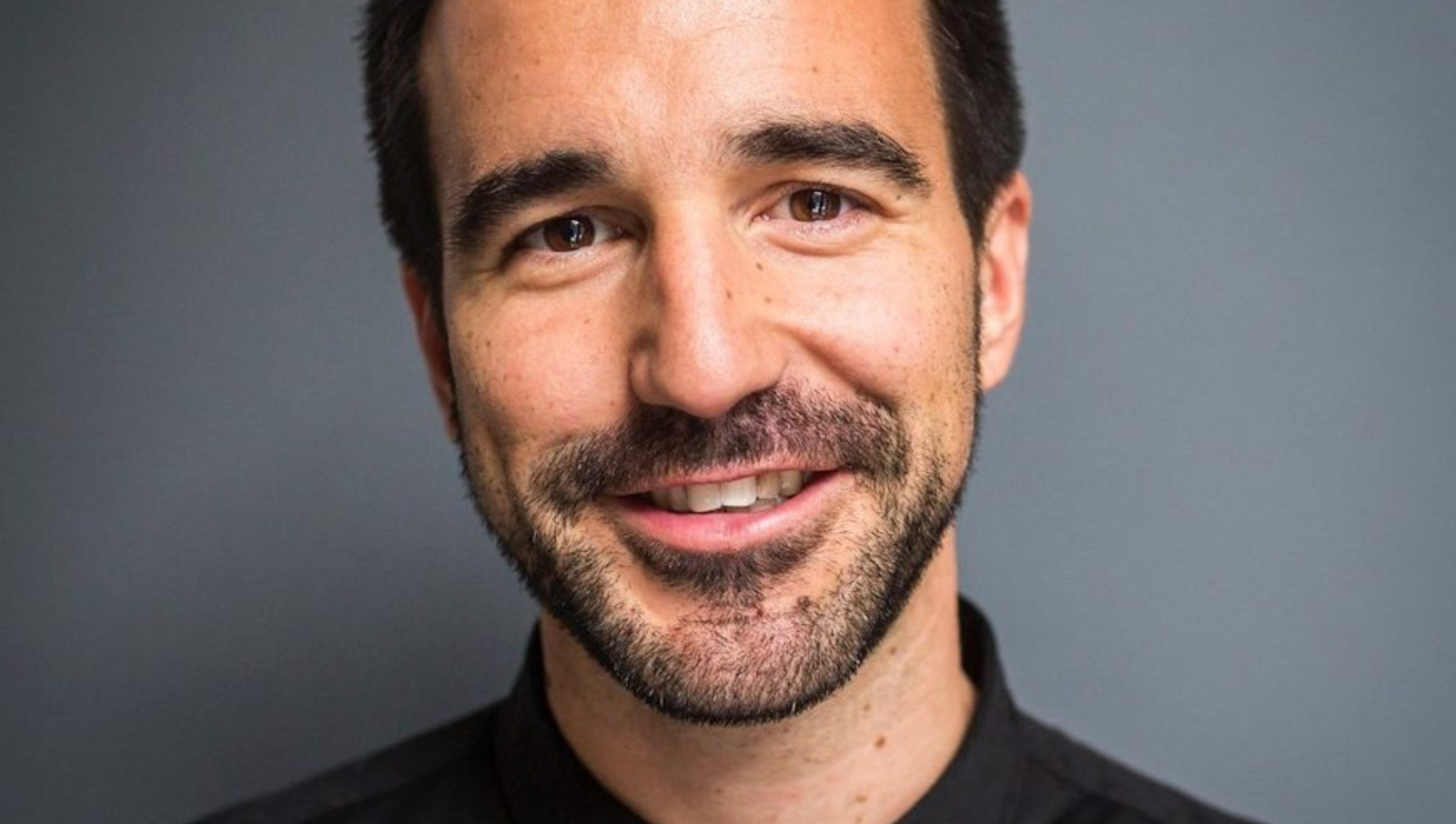
Tomas Chamorro-Premuzic
Tomas Chamorro-Premuzic is the Chief Talent Scientist at ManpowerGroup, a professor of business psychology at University College London and at Columbia University, and an associate at Harvard’s Entrepreneurial Finance Lab. He is the author of Why Do So Many Incompetent Men Become Leaders? (and How to Fix It), upon which his TEDx talk was based. Find him on Twitter: @drtcp or at www.drtomas.com.
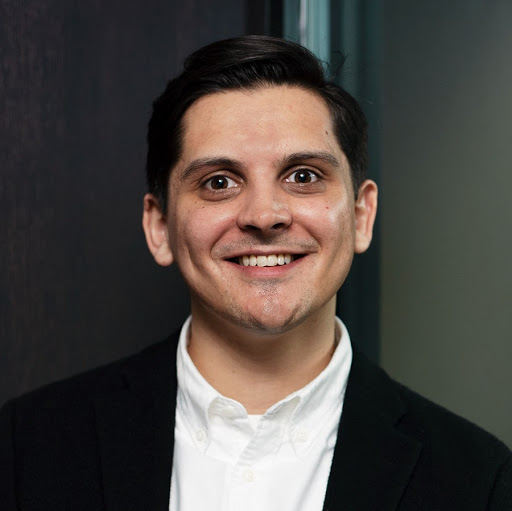
Reece Akhtar
Dr. Reece Akhtar is a co-founder and Chief Science Officer at Deeper Signals. He is an organizational psychologist and data scientist specializing in applied personality assessment and computational psychometrics. As a lecturer at NYU and UCL, he has published scientific articles on personality and machine-learning, talent management, and leadership. Previously he led product innovation at RHR International and Hogan Assessments Systems.
Recruit Smarter
Weekly news and industry insights delivered straight to your inbox.