There is currently a seismic shift under way in terms of how companies think about the fundamental concept of “value,” a long codified concept historically understood as more or less sacrosanct within the world of business.
Of course, business as usual is anything but these days, an evolution which is not only impacting how work gets done (and where), but also how that work impacts a company’s bottom line, too.
A half century ago, “value” was determined almost exclusively by tangible assets; think real estate, capital equipment or surplus inventory, among other decidedly mundane, but traditional, valuation drivers.
Every business school uses the concept of “widgets” to describe a generic product core to a company’s valuation; while these are intentionally interchangeable, the fact that “widgets” are inevitably some sort of physical property that can be easily valued at market rates shows just how entrenched this model has become, in theory and in practice.
Living Proof.
Today, however, this emphasis on these tangible, salable assets has become increasingly seen as something of an anachronism, a vestige of an increasingly obsolete manufacturing economy that’s long since been replaced.
Now, we have what’s commonly referred to as “the knowledge economy,” a historic shift whose implications have been extensively documented, but seldom quantified or completely understood.
That’s because in the past two decades or so, we’ve seen the rise of intellectual property like patents, brand trademarks or software algorithms as a core component of many business models.
These have proved much harder to quantify than stuff like surplus goods or machinery, but necessity, as they say, is the mother of invention, and markets have responded by determining, more or less, the relative worth of this revenue revolution.
Of course, just as we seemed to be getting a handle on the implications of these intangible assets, there’s another evolution underway in our understanding of value and the bigger P/L picture. Today, the meaning of company value seems to be shifting to something that’s neither a capital asset nor a proprietary product.
I’m talking, of course, about data.
Closer to The Truth.
In November 2016, Marc Benioff, the CEO of enterprise software giant Salesforce, a leader in the data-driven business revolution, spoke about this shift in valuation when commenting on the staggering sum Microsoft paid to acquire LinkedIn.
Benioff, contrary to most pundits, economists and analysts at the time, understood why Microsoft was willing to pony up for such a premium price.
Benioff’s remarks spoke to the possibilities of how a combined Microsoft and LinkedIn entity could easily be combined to create proprietary data streams, richer information and more actionable insights, effectively creating an ecosystem with an almost insurmountable barrier to outside competition.
The problem, according to Benioff, is that the current regulatory and reporting requirements don’t reflect the reality of a world in which data is not only one of a company’s most valuable assets, but for many, the single most important valuation driver.
Benioff remarked that Microsoft, long targeted by anti-trust regulators, was being given an effective pass from many of these bodies only because of a lack of precedent or protocol – and that by ignoring the intrinsic impact of data on a company’s bottom line, regulators were unable to properly do their job of policing monopolies or protecting consumer or investor interests.
Said Benioff:
“[Regulators] need to make a decision on what does this mean for the ability for companies to trade in data, and we’ve seen companies are acquiring companies to potentially create proprietary data streams to create barriers of competition, so if the US government isn’t going to look at that then another government will have to.”
It should be noted, of course, that European regulators traditionally are much more stringent than their US counterparts, and their previous successes at preventing Microsoft’s monopolistic monetization model was now being undermined in the absence of precedent or policies on data.
If there were indeed any anti-trust implications related to deals like Microsoft and LinkedIn, in short, there would be no way for oversight groups to know it.
Which, of course, presents a historic opportunity for many leading technology companies, who are already miles ahead when it comes to this new asset alchemy of spinning data into gold, ore or less.
Believe.
Google, for example, has an enviable and effective portfolio of tremendously valuable data streams; think of the power (and profit) inherent to the information being generated by products such as Google search, Android devices, Chrome, GMail and the rest of the rebranded “G Suite” of products like Presentations or Google Docs.
Facebook, similarly, has data on the preferences and proclivities of almost 2 billion monthly active users, a gold mine whose surface is just now being scratched – and sold. LinkedIn, similarly, is the world’s single largest repository of professional information, with over 400 million profiles (and counting).
How many of those profiles constitute active users may be a subject for debate, but the aggregate value of that information, obviously, is still worth tens of billions of dollars to a company like Microsoft.
Just because these tech behemoths are ahead of the curve doesn’t mean that they’re the only entities positioned to cash in on the shift to data for determining value. Every employer – and every in house talent leader – is likely sitting on a huge amount of data that, properly packaged, could be a huge asset.
The potential for TA to move from cost center to profit driver through data is significant, and every talent leader has a vested interest (no pun intended) in shifting strategic approaches to our understanding of why people are, in fact, every company’s greatest asset.
The only thing that this well worn cliche got wrong is how to tap into the actual value that talent related data really represents to a business and to its bottom line.
All I Really Wanna Do.
These various data sources can be bifurcated into more or less two categories.
The first is traditional employment data.
The second, and maybe the most overlooked (and undervalued), is non-traditional consumer or competitive data that can provide bigger business value while optimizing and maximizing the ROI on recruiting and retention, too.
Traditional employment data alone is obviously a huge area of opportunity for employers, when you consider the implications of understanding the aggregate impact of such basic information as:
- Every resume or application submitted directly to your ATS or system of record.
- Every job description for every role you’ve ever posted or req you’ve ever filled.
- Performance management and developmental data on individual employees.
- Retention data like tenure, turnover and reasons for attrition.
- Employee satisfaction, engagement and other structured survey data.
- Performance and relative ROI for different sources of hire, cost per hire and time to fill sortable by role, market or function.
- Public data on existing candidates and current employees.
- Salary information and compensation data for employees and applicants.
I can keep going, but you get the picture. Each of these seemingly simple assets can, in aggregate, help answer questions your recruiting team might be struggling with or provide insight into workforce planning and the bigger business impact of talent acquisition and management – something that’s never been directly quantifiable.
Until now.
Some of the most obvious questions that data should easily solve include such considerations as what resumes of high performing employees look like relative to the rest of your employee population or applicant pool, which job descriptions attract the most qualified candidates, or which recruiting channel provides the highest recruiting related ROI.
If you’re a recruiter, chances are you’ve struggled to obtain this information at some point in your career. Now, being able to answer these questions is not only a possibility, but an imperative.
Combine these traditional data sets with non-traditional data, and you’ll maximize the efficiency and efficacy of not only recruiting and retention, but overall employee productivity and performance, too – huge drivers of any company valuation that can now be actively measured and managed for optimal outcomes.
Some non-traditional data recruiters need to start incorporating in their approach to analytics include:
- The financial impact of the adoption of employee benefits, total rewards programs or other employee incentives.
- Leveraging consumer and B2C marketing data insights to know how to turn customers into candidates (and vice versa).
- The best place to retarget job ads or employer branding assets based on applicants’ previous online history, behaviors and interests.
- The impact of recruiting and retention on the relative performance of individual products and services, such as tying sales data to applicants to determine their relative impact on revenue.
- Leveraging customer service or account management data to understand which questions you should be asking new applicants, which objections and perceptions your recruiting team should address in their talent attraction efforts and how to optimize candidate experience (among other insights).
Companies that can combine these two distinct data streams and develop actionable analytics and more efficient, effective HR processes and policies are the ones that are the most likely to gain a competitive advantage when it comes to hiring top talent.
Companies like Facebook have already figured this out; the Palo Alto based employer recently determined that there was a strongly positive correlation between commute time and employee tenure, and started offering workers a $10k package to allow them to move closer to the office. The result is lower turnover and more engaged workers, as shown by the relative performance of those who took this inducement versus those who continued their current commutes.
There are a ton of examples like this, but this should show just how many easy ways there are to hack HR just sitting around waiting to be discovered. The answer to most of your problems (and prayers) can probably be found within the data you’ve already collected.
The key is understanding what issue you’re trying to approach, what data you need to build a business case and which repositories of raw data will have the requisite information you need to get the answers you need.
I know, that’s easier said than done. But the good news is, it can be done – and needs to be, honestly, if you want to remain competitive when it comes to recruiting the best and the brightest.
Here’s what you need to do to make this happen.
Take Me Home.
The biggest challenge most employers face when tapping into talent data is that they either don’t have any easy way of collecting and interpreting that data.
Even those few employers that have the technical capabilities to do so easily, however, very rarely also have the internal expertise to accurately interpret that data.
Among enterprise employers, less than 10% have a dedicated data analyst or data scientist on their HR or recruiting teams, which means that they have no way to make metrics meaningful, much less have the ability to really deep dive into these assets to proactively do any sort of forecasting, future planning or scenario analysis.
We already know math isn’t a strong suit for many recruiters, but it should be pretty obvious that the lack of internal resources committed to talent data doesn’t add up, and the opportunity cost of not having this capability remains higher than most employers can afford.
This isn’t an easy fix, of course; data scientists are hard to find, with demand far outstripping the fairly finite supply of quants out there; the few dedicated data scientists a company does successfully hire are almost unilaterally placed on a corporate strategy, finance or product team, not within the HR organization tasked with hiring these purple squirrels.
I encourage employers to think differently here; it’s very easy to quantify the contributions of a data scientist and build a business case for paying the premium price this talent commands by simply looking at opportunities for improvement, cost savings and revenue creation, even anecdotally or qualitatively, that would come from improved retention, higher quality of hire or knowing the relative performance and ROI of various recruiting channels and sources of hire.
The Sonny Side.
Individually, each of these outcomes has the potential to be a game changer in talent management and attraction.
Taken together, the impact should not only lead to better hires over the short term, but increased revenue over the long term, too.
And that’s the bottom line.
Every applicant, even the ones who don’t meet even the most basic of basic qualifications, represents a potential treasure trove of data, not to mention the incredible amount of information we have in HR on successful candidates and current employees that we collect, but for some reason don’t utilize in any meaningful fashion.
This is not only a miss, but it means that in a world where value is increasingly intertwined with data, recruiters and HR organizations who don’t have the ability to interpret or leverage data are essentially worthless.
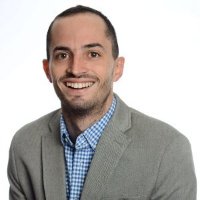
Recruit Smarter
Weekly news and industry insights delivered straight to your inbox.