Nearly every vendor in the HR Tech & Recruitment space claims to be using artificial intelligence. If you product doesn’t have AI, it is a clever idea to dilute the impact of tools that do by making AI into a buzzword and give rise to claims that AI doesn’t exist. But there are several tools that do utilise artificial intelligence to enhance recruiters. How do you assess who has AI? How do you assess how good it is? Here are five points, that help evaluate AI systems.
- Human-Centric Functionality
- Built by experts
- Transparency by design
- Putting the user in control, not the system
- Mitigating bias
Human-Centric Functionality
The promise (and reality of AI) is that it is designed on human behaviour.
One of the big differentiators between machine learning and automation is teaching a machine to do something with similar discretion to an actual person versus teaching a computer to do a repetitive task faster than a person can.
Keyword searches are a good example of automation. No matter how advanced the search algorithm, it isn’t machine learning. Semantic search, search based on natural language understanding, is an example of machine learning:
A system looks to match key terms from the opportunity across tens-of-thousands of resumes. Once it has found those terms, it looks for supporting language surrounding those terms that give the system context. That context is critical to understanding ‘Java’ in a barista’s resume versus ‘Java’ in a software developer’s resume. In other words, can it scan the resume like an expert recruiter? Does the AI understand and replicate for example, how a recruiter thinks? What a recruiter thinks? What the interactions between candidates and recruiters are? What the interactions between recruiters and hiring managers are?
Key question: How does your system make assertions based on information in a sentence?
Built by experts
It is important to ensure the system you are using is an ‘expert system’.
Firstly, is it trained by experts. That isn’t just important for training the AI, but also for developing a user interface that is easy to use, doesn’t require much learning, and fits neatly into the recruiters’ workflow instead of trying to alter or replacing it.
Secondly, has the taxonomy been built by expert recruiters or by linguist experts?
Have the tools been built from the ground up – from tokenizing to part of speech tagging and word embeddings? The best tools have been trained on resumes and job opportunities, not romance novels or news articles and their taxonomies are built by expert recruiters, not linguistic experts.
This is very important as resumes and job opportunities present unique challenges from a language processing standpoint. Inconsistent formatting, a mix of semantic and non-semantic content, a wide range of industry/job specific terminology, and the temporal aspect of experience are all challenges that require purpose built solutions.
So buying an off the shelf product that has general AI is not good enough and will end in disappointment. Most natural language processing doesn’t sound very natural. Even Google has called out the underlying tools as not accurate enough to build complex systems on.
It needs to be an expert system, modelled on recruiters, trained by recruiters using recruitment related data and building tools that are based on the intricacies of recruitment.
Key questions: Who and with what data has the AI been trained?
Transparency by design
Much of machine learning suffers from a black box problem. That means as models become increasingly complex, it becomes increasingly difficult to explain why a given outcome occurred. The importance of each data point in the decision-making process isn’t easily explained. When it comes to hiring, that’s a violation of both American hiring regulations and EU’s GDPR.
It is better to implement linear models instead of black box deep-learning. This approach requires smaller datasets and less learning time. It has the added benefit of being completely transparent.
Knowing what each data point is and how it’s weighted matters. Users trust what they can see and agree or disagree with. In the minority of cases where the system makes a mistake, transparency allows the cause to be obvious. When we talk to users about the system showing them a bad resume, they usually say, “But I get why it made that mistake.” Right or wrong, the answer makes sense.
It is important that users understand how the algorithm works and results are achieved, to create trust and eradicate concerns about any future regulations.
Key question: Can you prove that no decision was made based on data points which could result in age, gender, or any other protected class discrimination?
Putting the user in control, not the system
As mentioned in the last point, much of machine learning suffers from a black box problem. That means as models become increasingly complex, it becomes increasingly difficult to explain why a given outcome occurred. Humans fear that they can’t control the system, but the system controls them. We believe it is important to build systems that are built for humans, which means the system needs to listen. In data science circles it is called ‘reinforcement learning’.
Outstanding tools put the user in control, not algorithms. They give users the ability to sidestep the algorithms by putting a greater emphasis on their decisions and activities, such as candidates they select and reject. The system then learns from user feedback making each search more accurate than the last and personalizing candidates shown based on user selections, not the average selection. Let the user teach the system with every interaction.
Key question: Is the user in control or the algorithm?
Mitigating bias
Unconscious bias comes in two forms: biased data and biased parameter tuning/feature engineering.
We are in recruitment. We are dealing with people. People are biased. Every job description written by a recruiter is a reflection of their reality and desires, so is every resume. The question isn’t “Is there unconscious bias?”, but “how do you deal with unconscious bias?”.
Here are some steps to mitigate the impact of those biases:
- Diversity of source data (resumes and opportunities) helps ensure no single data gathering bias impacts the learning.
- Avoid topic modeling or any other algorithm that averages document contents to make a decision.
- Focus on terms and look across thousands of term instances to determine what to search for beyond just the terms in front of you.
From a feature engineering standpoint:
- Don’t review any protected status feature nor allow any predictor of protected status to fall into models.
- Use linear models. Each feature is easily audited by looking at the results. Any undesirable feature is self-evident by a review of multiple results and can be overridden by the users’ selections/rejections.
Key question: How do you deal with unconscious bias?
These 5 questions allow you to filter out any charlatans early on. As a next step, I would insist on a trial, so you can use the system and experience the performance and results for yourself within your company’s and industry’s context.
Authors
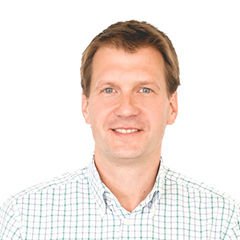
Felix is the CMO of Pocket Recruiter, an AI based expert sourcing tool, and an active participant in the HR tech startup scene. Before that he worked for Jobsite.co.uk since 1999 and played a significant role in the growth of the brand and evolution of Evenbase. A leading commentator on digital marketing, social media and the future of recruitment, Felix’s career has included marketing and journalism roles for leading brands and media organisations in Europe. He holds an MSc in Marketing from The University of Glamorgan, a DipM from the Chartered Institute of Marketing (CIM) and is recognised as Member, CIM.
Recruit Smarter
Weekly news and industry insights delivered straight to your inbox.