Algorithms make a lot of decisions for us, whether we know it or not. They decide what ads we see, what Youtube video plays next and a lot of the experiences we, as digital natives experience today. But what interests me the most is that algorithms the mind uses to make decisions.
Take the election season, for example. As a data scientist, I realize that who we choose in the first place is the consequence of a thousand different inputs from media, relationships and demographics. We’re reminded of the power in our decision simply by logging into our social media channels every day. Despite common sense and political courtesy, you’ll find a lot of people trying to convince each other of one thing or another but even more around election time. I’m not sure why people insist on talking about their views on policy and ideology, but nevertheless it’s prime season for political wars.
Media conglomerates and candidates with too much money are even creating quizzes that help you see how your beliefs and values align with different politicians and their voting records. They make a match based simply on your answers to questions. While there are some obvious indicators for one party or another, actually deciding the right decision based on outcome isn’t so easy. Even with all of those dots of data on certain policies, the bottom line is that we make our decisions based on how we feel about the person, not based on the metrics and inputs. Just like we do about people we hire.
Voter Fraud: Trusting The Unpredictable
The unpredictability of making the right choice in hiring and in elections is a data point too. When we’re uncertain of the outcome, we incrementally become less trusting of the system as a whole. When we can’t justify why a series of points add up to a concept we disagree with, we inevitably point to the unpredictable. The outliers.
But the numbers don’t lie. They can’t. Numbers are inputs for an output and the people creating the formula are the ones that maneuver and twist the information to make the outcome say what they want. However, people are highly unpredictable and “always” isn’t safe territory, even for numbers. Sure, if you’re predicting weather patterns and likelihood of winning the lottery – the numbers are pretty straightforward. Not so much for people.
That’s probably why teams show such disdain for the metrics behind people because they always have an outlier, someone they can point to that doesn’t fit the mold, that’s not fitting into the equation. And when it comes to a data point, it’s easy to cut. When it comes to people? Not so much.
There’s so much more here than a decimal and a digit – it’s a life, a personality, a relationship. Even though hiring with an algorithm has shown promising results for increasing the diversity and quality of your candidate pool while simultaneously decreasing your hiring costs, like any new recruitment technique, it’s been met with skepticism. And any potential benefits of hiring with an algorithm are going to be moot if you can’t convince people of its value in the first place.
Voter Registration: Getting Buy-In On A Big Bet
Hell, we still haven’t convinced everyone in hiring that they need to be measuring anything so it’s probably safe to assume it will take more than proof to build trust in the value of data driven decisions. People just don’t trust the numbers – almost as much as they don’t trust most politicians. In my experience, it takes a little more than a simple post or persistence. Here are 4 ways I’ve used to get buy-in for hiring with an algorithm from a reluctant team.
- Use statistics to get buy-in for hiring with data
For the analytical, facts and figures members of your team, hit them with the stats. Research confirms that this works. Presenting information in a graph decreases people’s initial resistance. Here’s a good stat to convince them: a survey of 100 recruiters found that 91% of them said adopting technology has made their jobs easier by reducing their cost per hire. Hiring with an algorithm is basically adopting technology to reduce cost of hire and improve quality of hire.
Here are some more stats for you: An analysis of more than 300,000 hires found that employees who were hired using an algorithm based on a test that assessed personality, intelligence, and job fit stayed on the job approximately 8% longer and performed just as well as employees hired by human recruiters.
- Use storytelling to get buy-in for hiring with an algorithm
A statistic (ironically) that often gets quoted is, “After a presentation, 63% of attendees remember stories. Only 5% remember statistics.” So for the team members unconvinced by the numbers, tell a compelling story about hiring with an algorithm. Make your storytelling even more persuasive by using social proof: it’s easier to convince someone to change their mind when you provide an example of how someone similar to them benefited.
For example, SAP started using an algorithm to recruit sales people, which saved their recruiters hundreds of hours in manual resume prescreening and saved them more than $370,000 in annual costs. If SAP doesn’t resonate with your team members, find a testimonial or case study that does.
- Start with a small test to get buy-in for hiring with an algorithm
Some members on your team might be particularly risk averse. Or maybe they’re skeptical that hiring with an algorithm is just the latest fad. So start with a small test . This makes it both relatively low risk and low investment. For example, perhaps you test by adopting the technology for a single role during a specific time frame. It’s ok to insist that partners offer time for testing before you put strain on your HR or IT departments by implementing a large scale tool that doesn’t give the outputs you need.
Your team can assess the quality of the candidates, the time taken to hire, and other recruiting metrics using an algorithm versus more traditional methods of hiring after, for example, 45 days. Once you can quantify and demonstrate the ROI of hiring with an algorithm, even the most skeptical member should be won over.
- Talk to your team to get buy-in for hiring with an algorithm
Probably seems pretty straight-forward but a lot of Type A, data people forget to just talk to the team. Sometimes your team is resistant to change simply because it implies that what we’re currently doing is wrong, not because what you’re doing isn’t right. Listen to your team’s concerns and objections. What is it that they’re worried about with using an algorithm to hire? When you’re able to communicate that you understand the problem your team member is trying to solve, he or she becomes open to hearing your ideas for a solution.
Here’s a case. Maybe the biggest pain point for your team member’s job is time wasted prescreening unqualified candidates. Replacing this inefficiency with an algorithm should be welcomed with open arms. Or maybe their top priority is increasing the diversity of the candidate pipeline. A study of more than 150 companies found that companies that hired using an algorithm based on a personality assessment had more diverse workforces. Once you figure out what problem your team member is dealing with, you can tailor your messaging on how hiring with an algorithm fits their needs.
You Choose: The Bottom Line
Data can’t work without the right inputs and buy-in from people who believe in the cause. No matter how convincing you think you are, ego leads people to think they’re always right.
It doesn’t help that traditionally, hiring has been considered an art involving human judgment. When you ask someone to remove their idea from the process, we also have to consider the conversation and justify why we’re making the change.
The bottom line is that hiring with an algorithm is more of a science that has the potential to increase the quality of your candidate pipeline while decreasing hiring costs. If that doesn’t hook a recruiting team, I don’t know what will. But I’d say it starts with a better conversation.
Ji -A Min is the Head Data Scientist at Ideal, a talent marketplace for sales professionals that helps recruiters automate candidate sourcing, prescreening, and shortlisting. Using her Master’s in IndustrialOrganizational Psychology, she conducts research on how to best source, recruit, and hire salespeople. You can connect with her on LinkedIn here.
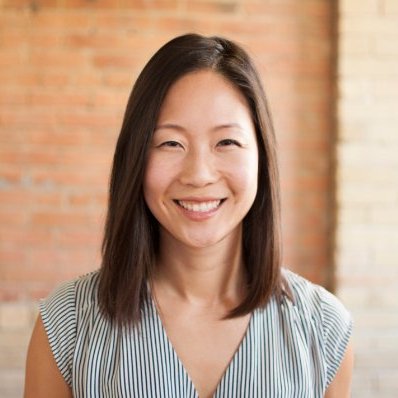
By Ji-A Min
Ji-A Min is the Head Data Scientist at Ideal, AI for recruiting software that automates time-consuming tasks such as sourcing, screening, and messaging. She has a Master’s in Industrial-Organizational Psychology and her interests include data-based recruitment, HR tech, and diversity. Follow her on Twitter @ji_min or connect with her on LinkedIn.
Recruit Smarter
Weekly news and industry insights delivered straight to your inbox.