Storytelling About Moonhub With Nancy Xu
Welcome to the Use Case Podcast with William Tincup! Today, we have Nancy Xu from Moonhub, and we’ll be talking about the use case or business case for why their customers use Moonhub.
Moonhub is a startup that aims to change the way companies find job candidates with a real-time people search powered by AI. The platform finds billions of data points of individuals from the public web, and then builds an AI layer on top to analyze all that information. With it, the company helps to unearth hidden candidates – qualified workers that wouldn’t be found using traditional recruiting methods like keyword searches – so companies around the world can hire the best talent.
Their story of innovation and adaptability in the face of adversity is sure to inspire others. They understand that building a successful startup in today’s ever-changing business landscape is no easy task.
Give the show a listen and please let me know what you think.
Thanks,
Show length: [28] minutes
Enjoy the podcast?
Be sure to check out all our episodes and subscribe through your favorite platform. Of course, comments are always welcome. Thanks for tuning in to this episode of the Use Case Podcast!
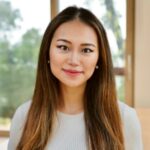
Nancy Xu
founder, ceo at Moonhub (people search powered by llms) 🌙. Moonhub is building the world's largest real-time people search engine, powered by AI. By analyzing billions of public and private datapoints we help teams scale and discover the best candidates for any job opportunity. Engage a long tail of diverse, under the radar candidates brought to light by Moonhub.
Early-stage Investor -- love B2B, AI, consumer, healthcare companies. -1 to Series B ... Would love to hear from you if you're a determined founder with a problem you’re passionate about + vision for the world.
Never lose sight of what matters to you. I grew up the child of immigrants and love working with people who feel they have something to prove to the world.
FollowStorytelling About Moonhub With Nancy Xu
William Tincup: [00:00:00] This is William Tincup and you are listening to the Use Case podcast. Today we have Nancy on from Moon Hub and we’ll be learning about the business case or use case or prospects and customers use for purchasing Moon Hub. So Nancy, would you do us a favor and introduce both yourself and Moon Hub?
Nancy Xu: Hi William.
Thanks for having me. Sure. Absolutely. Hi there everyone. I’m Nancy Shu. I’m the founder and c e o of Moon Hub. [00:01:00] I, uh, live out here in the Bay Area. That’s where I’m calling you from. And, uh, I’m a computer scientist by training. I was previously a, at Stanford as a computer science PhD and an m mba and, uh, left to start this company.
And at Moon Hub what we do is we build ai, um, for recruiting. Um, and our goal is to help fast growing companies, like hopefully many of the, the folks who listen to this podcast, um, represent and, and help them scale their teams with our technology. So
William Tincup: an mba, PhD, Stanford. Super easy to get into both those.
I’m imagining you just kind of went by, went to Palo Alto, just kinda went by campus, filled out a couple forms and Yeah, not a problem. Um, actually I was, I was on campus last year at such a beautiful campus, uh, Such a beautiful, so you left that to do this. So, you know, um, but I do wanna start with kind of, uh, a few things about [00:02:00] AI for recruiting.
So, you know, normally when you have the conversation on, it’s, it’s, uh, it’s about automation. It’s about let’s get the lower value task. Let’s automate those things. Let’s get AI in there. So why don’t we start with kind of your initial, kind of the, uh, thesis of what you want, where you saw kind of the biggest EF inefficiencies and where you could apply ai, machine learning, and lp et cetera, all the things that you would apply.
So where did you, where’s, where’s the first thing that you wanted to kind of eliminate or, or make more efficient is probably a better way to think about.
Nancy Xu: Yeah, I, I love it. It’s a great question. Um, can I, can I tell you a bit about how the company started? A hundred percent, yes. Awesome. Alright. Well, you, you know, the, the story behind Moon Hub is actually quite simple.
I, I’m a first gen, first generation immigrant to the United States, and I feel like throughout my life, one of the biggest gifts that I’ve been given, um, is access to opportunity. I [00:03:00] fundamentally believe that one of the best things and the greatest gifts you can give to anyone on this planet is access to opportunity.
And so, you know, I, I’d gone to Stanford for, for my undergrad as well. And over the course of the last couple years, I have had the chance to mentor some incredibly talented individuals and, uh, many of them. When they, it came time for them to look for a job, it would come to me and ask me for help. Um, getting introduced to companies and because I’m out here in Silicon Valley, oftentimes they wanted to be introduced to startups.
And, um, what, what you would see is these folks who, because I had worked with them, I knew how talented they were, but yet they weren’t being reached out to by many companies and, and oftentimes many companies that they ended up joining and. The, when you dig deeper, you realize that there’s this huge, um, inefficiency in the way that individuals.
Uh, get, get access, um, to, um, [00:04:00] opportunities. And a big part of that is because of our current technology system. So was some of that was,
William Tincup: was some of that bias, uh, Nancy, as you kind of looked at it, it was, some of that was, uh, people’s backgrounds or where they came from or gender or any of, any of those types of things?
Or was it just,
Nancy Xu: or I mean, it could come from, it can be personal bias. It can. Sort of just like top of mind, like bias in terms of the companies, you know, the schools you highly of. Um, but what, what you look at today is most recruiters, like they, they use these very basic search tools to look for candidates and Right.
Um, and my fundamental philosophy and belief is that, um, 10% of the candidates that are a great fit for any opportunity are actually being reached out to and engaged. And the remaining 90% are never seen by any recruiter looking for that, helping do the search for that role. Wow. And the reason is because if I’m sitting there and I’m like, you know, I’m, a lot of, a lot of companies say they wanna find like machine [00:05:00] learning engineers.
It’s like ai, it’s the hot new thing and, and they’re trying to find these individuals. If I type in the keyword ml, On a, let’s say LinkedIn, right? I’m not gonna find the person that’s mentioned. NLP engineer or the person that says machine learning expert title. And similarly, if I look for, I want someone with a skillset Python, and I look for that key word, I’m not gonna find the person that mentioned pie torch, which is like a, you know, a variation of Python that AI people often use.
Or like, I’m not gonna find the person that mentioned chat, G P T or large language models or what have you out there. And that is like super exciting in this space and all skills that someone who is a. Machine learning engineer would have, but you just never find them because there’s keywords exist. And so, um, for us, really what, what I believe, um, you mentioned sort of automation earlier, I think AI has a tremendous potential to not [00:06:00] only help make, um, existing workflows more effective or efficient through automation and working with the real people doing those jobs, but also actually broadening the landscape.
Opportunity and possibility of what we think of as recruiting. Um, right. Like what if you could have like a, um, what if every hiring manager on this planet could have access to a conversational system who would just serve as their recruiter? You know, what if like that 90% of individuals we’re all being contacted now and, um, oftentimes they’re more.
They don’t have access to the same opportunities. They’re being reached out to by 10 x less recruiters, and hence they’re more willing to engage about any, um, anything that you put in front of ’em and, um, you know, et cetera, et cetera. Uh, but that’s, that’s really where I think the potential of AI is in in the future.
So,
William Tincup: so a couple things along those lines. Where do we, where right now, where does Moon Hub, uh, where do you connect, where do you sit [00:07:00] in the workflow, uh, for your clients right now? Like, where is it a separate application? Is it integrated into, uh, ATSs or other, other, uh, other types of tools? Like, just give us kind of an idea of when they, when they use Moon Hub, how are they using it?
Where are they using?
Nancy Xu: Yeah, we’re, we’re the recruiting partner for our companies. Mm-hmm. And so we recently launched what we call Portal. And the way you can think of portal, it’s like a, is an ATS for companies. And, um, right now companies come to Moon Hub with a, with a role that they’d like to hire for.
And then they can work with us either on a per placement basis or as on a, um, a retained recruiting basis. But in either case, they go through portal, they post a job, they get a dedicated in-house recruiting expert on the Moon Hub team who’s working with our AI technology to help them find candidates for that role, um, move [00:08:00] candidates through the pipeline, and then ultimately, um, help, uh, candidates get offers and, um, for companies to hire those.
William Tincup: So, so right now we’re, uh, well, it’s obviously technology infused service, right? So the, the tech is the, the underneath it under makes the machine go. Um, but it’s you, it’s a services layer where kind like not staffing or s p or rpo, but people can come to you and say, we have this. And then you’re, you know, Obviously the technology.
You’re, I was about to say your people, and I’m like, ah, okay. The technology is then, uh, finding ’em candidates. Um, so, so how do, um, you know, again, what I love about this is again, Most firms don’t have kind of a, that, that that secret engine they use other, yeah, they, they use, again, some of the tools that you mentioned, you know, LinkedIn recruiter, seek out, hire easy sourcing tools to find people.
Uh, but you’re, you know, this is, this is much different than [00:09:00] that in that, uh, you take their job description and then you’re trying to. Better matches and it, it gets, I would assume that it gets smarter over time, uh, as they, as they bring in a job description,
Nancy Xu: et cetera. Yeah, exactly. And I think the, the really exciting thing here is, um, when we first started the company, I was the recruiter on the team.
I was like, you know, I’m, I’m a, I’m a computer scientist by training. Um, but I really do believe that recruiting is like one of the, uh, is such a, such an important goal you can play in any company. And, um, I’ve, I spent months doing what, like recruiters and off, and probably many people on this podcast do in their daily jobs and I.
You, you get into this role thinking that you’re going to talk to candidates all day long and really have an impact on their lives. And I think, um, you really do get that. But imagine if you could spend your entire day doing that and [00:10:00] not having to worry about like the sourcing components, all those emails that you sent and.
Um, and that’s really what we’ve built the system to do, is to enable recruiters and enable hiring managers to have that, to, to get time back and to be able to spend their time doing what they, what they love.
William Tincup: So, so questions that buyers should ask you. Well, I want to, I’ll usually do this towards the end, but I wanna ask this now.
Yeah. Is cuz this is, this is, this is new. Um, cuz there’s a technology component to it. Um. Most, I would say the historically staffing an rpo if, if they wouldn’t care how you got the candidates, you know what I mean? Like that they wouldn’t even ask the question. They’re just like, okay, I want, I want, I want six of these.
Um, but, but I would assume that part of your relationship was, is showing ’em the demo, showing ’em technology, showing ’em kind of how you work and how it’s different and better. So what, what questions should they ask? Cuz this is a little bit different than, than, than things that they’ve historically bought.
Uh, what questions should they
Nancy Xu: ask? [00:11:00] You should ask the same questions you would ask, any recruiting partner you want to work with. And I can tell you our, our average time for a client to meet the candidate that they end up hiring for one of their open roles is about three weeks. Wow. For most other solutions, it’s months.
And we do this because of our technology and also because we have great in-house recruiters too, right? Like we do a lot of, we invest a lot of our resources in, in, in finding the best individuals and really helping make sure that if you’re trying to find a marketing manager, the person who did the go-to-market hiring at some of the best companies out there is helping you on that job.
Um, and we do have a really strong belief in quality as a company. Um, We, about one in four candidates that we introduce to a client end up actually getting an offer at the company. And I think there’s, I have yet to hear of another [00:12:00] agency that compares on that metric. And, um, for us, that’s something we’re very proud of because at the end of the day, we wanna be a partner.
To your company and to help you be a talent superstar. Right? And like, it’s not helpful if I’m sending you a hundred candidates and you have to spend all your time fishing through who’s actually interested, who’s even like available and on the market, who would even consider the role or, and who’s just like there because they wanna learn about the company but would like reasonably not actually, you know, even, even consider an offer.
And so we do a lot of that like work for, for our partner.
William Tincup: So tell me, uh, a little bit about the, the, they’re uploading a job description cuz you know, there’s a lot of historical baggage around job descriptions and, and, uh, biases that, that kind of happen either, either knowingly or unknowingly, uh, subconsciously or consciously, I guess.
So what do, what do you, as you look at job descriptions, you’ve probably studied it, what, what do you look at that there and, and has the [00:13:00] technology. The secret sauce, of course, but how does it look at job descriptions and make a more refined search out of, you know, garbage, generally speaking, that comes in.
Nancy Xu: Hmm. Yeah, it’s a, it’s a, it’s a great question. One of the things we do is, um, We’re building a conversational system inhouse. Mm-hmm. Um, and I should also caveat all of this with, like right now, we provide this, the sort of what we call the managed service to our customers. Right. But later this year, we’re actually going to start releasing some of our AI features directly, um, for people to use.
Oh, cool. And I’m, I’m super excited about that. I think it’ll really. Both, um, how folks, um, you know, existing recruiters approach recruiting, but also the whole landscape of like, if I’m a hiring manager, like I can just use this directly myself, right? And I’m really excited. Um, so, so how does it work? Like if [00:14:00] you give me a, if you give our system a jd, it’ll actually proceed to have a conversation with you to clarify elements of the jd.
So, um, it, it might say, oh, I saw your JD based on this. Like, I know you’re hiring machine learning engineer. Um, oftentimes. Skills that are relevant or languages might be PyTorch, TensorFlow, um, et cetera, et cetera. And then it would actually sort of work with you to fine tune what it is that you’re looking for.
Oh, cool. And then get an understanding of the role. And then based on that information, run a search against our, um, our database, and then show you the profiles that I think are relevant. Um, part of this is also just saving you a lot of time in running the search and knowing what to search for, because the truth is, most recruiters, they haven’t been machine learning engineers themselves.
Right? Right. And they shouldn’t have, they, like, they should be [00:15:00] able to do their job without having been one. Um, they, they may not know, like when, when someone, when they’re hiring manager tells ’em, Hey, find me someone who works at. Another super top machine learning company with like a great team that does like large language models.
Like as a recruiter, you may not frankly know what those companies are. Our system would go in and actually you’re
William Tincup: being, you’re being kind, Nancy, you might not know is more of a, yeah, come on now. But that’s fair. Appreciate that.
Nancy Xu: Right? Like you’re gonna Google that. So think about all of it. Google on a day.
And instead of having to Google it, our system will just be like, here’s a search. And I’ve already applied the filter for you of like, these are a hundred companies that you should be looking at. Based on what you’re, what you told me. And it’ll populate all a hundred of those four years. Oh, I like
William Tincup: that On a couple levels.
But I want to ask, uh, when it services the profile, so after you kind of [00:16:00] run the initial search, did, do, do, does it get tighter in terms of they like this, uh, or they give you context as to that’s not a good candidate, this is a great candidate. Like does it, how does it learn? How does the system portal learn more?
Uh, preferences is such a loaded word, but has it learned what they want out of that, out of that kind of that
Nancy Xu: candidate? Yeah. So currently, if you were to work with Moon Hub today, um, your first interaction would be a 30 minute onboarding call with your recruiter, right? Would sort of go through this process and fine tune the search with you and do what we call calibrate.
In the future, what we see this looking at like is actually you are just talking to, um, we call our conversational agent Lunar, but lunar directly. And, um, lunar will have this conversation with
William Tincup: you and it just keeps tightening. Uh, it
Nancy Xu: just keeps, yeah, exactly. And so you can imagine, you know, you, [00:17:00] you converse with Lunar.
You see in our search, um, page the search results and what filters and. Features were taken into account to produce those search results. And you can give it feedback. Say, Hey, this profile not good because we’re not looking for a manager. We want an ic. Right.
William Tincup: And it’s the context so that it learns the context of why not just an up, down or Yes, no.
It learns the context of why they said yes. No. Yeah.
Nancy Xu: So it’s not a thumbs up, thumbs down. It’s really the core of what we build is a lot of understanding natural language. Right. And use. Human language as the interface versus like boo or some kind of code and really taking the full flexibility of natural language and understanding that.
I love
William Tincup: that. I forgot to ask you at the beginning, do we, are we, we, because we’ve talked about machine learning, uh, engineers are, do we have a specific focus? Focus in terms of, uh, of the. [00:18:00]
Nancy Xu: Yeah, it’s a great question. So, um, moon Hub currently works, um, we work across functions and I wanna highlight that we serve companies of all sizes, right?
So we’ve, with early stage startups, large public companies, fast growing growth companies, um, Public sector companies, um, you name it. And the, the sort of defining quality of all the companies we work with is one, um, they are hiring and two, they not, not
William Tincup: fake jobs on indeed.
Nancy Xu: They’re, and they, uh, generally are more innovative, so willing to sort of engage with technology.
And then lastly, we, most of the hiring we do is in sort of what I call like the mid-level ic. Um, Uh, uh, section of, of roles. So we don’t do a lot of exec [00:19:00] hiring. I think there are great exec recruiters out there who can do that better than we do, and we’re very happy for them to do the exec hiring. Um, we really shine if you’re looking for that marketing manager or that backend engineer or that like, um, you know, even, um, a, an IC on the sales side, like that would be the, the place where Moon Hub would really be able.
I
William Tincup: love that. I love that. So let me get your take on ethical AI and audited AI and the, and the reason why I wanted to ask you this cuz A, you’re an expert, but b um, you know, I see, you know, lots of conversations around ethical ai. So I just wanna get your take on kind of as you’ve built this, How do, how do you, how do you and your team kind of intend to kind of make sure that it stays on path or you have to, you know, obviously have to make changes to the algorithms, et cetera?
Uh, cuz you know, hiring is, I asked somebody the other day, I’m like, what’s the difference between preference and bias? And her answer was so [00:20:00] great. She said, uh, well, preference is how you justify bias. And I thought, whoa. All right. I mean, it’s, I mean, cuz one of them’s, when you say preference, people are like, oh, okay.
Yeah. When you say bias, it’s loaded. Like both words are loaded, right? They’re both loaded. Um, but I wanted to kind of get your take on kind of like, okay, you obviously set up, uh, the initial, your team set up the initial algorithm and you. You’ve probably got a process in place to make sure that you’re doing everything as ethically as possible, but you know, it is technology.
So, you know, how do you look at ethical ai and also kind of the side to that, how do you audit your ai, how do you plan to, what’s your, what’s your
Nancy Xu: take on that? I am, I’m glad you asked this question, William. The we care about, Fairness and we care about, um, making sure there’s limited, [00:21:00] if no bias in the system as much as possible, and one, as a company, we really believe that our core mission is to enable access to opportunity for as many people on this planet as.
And we are a very impact driven company. And for us, we think that by building these AI solutions that help more people be seen for who they are beyond the four keywords that pop up the most in their profile is going to help a long, um, tale of, uh, diverse people who have oftentimes been forgotten or been, um, Under underrepresented Frank, quite frankly, in, in this system, uh, be seen.
Right, right, right. And, uh, but concretely to your point of how do we think about [00:22:00] AI as a company, so, um, we. Have made some deliberate decisions in the product, um, to reflect this. So one is we don’t, um, we could easily have this like whole conversational system. We show you search results and we don’t really explain to you why you’re, you’re seeing ’em.
Right, right, right. And so one thing, explainability in the system, we, we made a very conscious decision to, um, show you the sort of, To the search results that you see and allow you to fine those inputs yourself so that you as the end user, um, have a say in determining how you want your, your search to be represented and the types of people that you see.
And then similarly we do, I think within the AI space, there’s a lot of conversation around with these more recent models that have the ability to basically say whatever mm-hmm. Um, to make sure that our information is actually factually correct and granted. Right. Right. [00:23:00] And the, the, to the latter point, we address this by one backing up our, a lot of our, um, the things that our system says with.
And making sure that, um, for many of our responses in the conversational system, we don’t actually let it just like, say anything. We, we actually have it generate a question that it asks on our data set, gets the results from the data itself, and then present it to the user. So a lot of the information we share is like actually backed by data as opposed to, you know, so and so said on the.
William Tincup: So, and I know, I know you probably could ask this question, but I I, I want to ask you, uh, so outside of that, first of all, you answered that wonderfully, and I, I know the audience is, is gonna love that. What if, what if a client is, you know, this is now the opposite of, of, of bias, right? This they’re looking [00:24:00] specifically to, uh, d.
Right. So they, they recognize, they, they have a weakness, let’s say. Um, and they, they specifically would like, um, female, uh, engineers, let’s say machine learning engineers go real, real tight. So, so it’s almost like they really, they, they, they want to be biased. It sounds, sounds weird to say it like that, but, um, there’s, there’s, they, they, they’re actually, they recognize there’s a problem.
Their d e i folks recognize there’s a problem and they’re look specifically. For candidates that fit a specific, um, uh, a agenda that they’re trying to solve for. How do you, first of all, how do you deal with that? I mean, how do, how do you, I mean, cuz you know, on one side of it’s like, Hey listen, we wanna give everyone a fair and equal chance.
You know, just let’s anonymize the photo, let’s anonymize all this stuff on their resume. Let’s just make it about skills and expertise, potentiality like that makes sense. Until you run into a leader that actually is having a problem, that [00:25:00] they want to recruit very specific people. That’s that, that, that, that the anonymized actually is working against their interest.
So how do you, first of all, have you had conversation? I don’t wanna make up something that’s not there. Have you had conversations with clients about this?
Nancy Xu: I think diversity is a big issue amongst a lot of companies, and it’s something that’ll many and I, I, I think rightfully, many talent leaders should be thinking about. The, the way we approach this is we, we always wanna make it so that any information we provide that is. Um, re related to diversity, it’s used in a positive way.
So for example, you could not in our system filter if like someone is a, is a identifies or is is male and then only, you know, reach out to those people. Like, we would not support that in our system. Right. And, um, and, and I will say that there [00:26:00] are ways to work with what people self-identify as. Um, Publicly and use that information, um, to the advantage of you as a company or someone who really wants to promote diversity.
So for example, people who have said, oh yeah, I’m part of like a woman in computer science organization, or, these are sort of the types of signals and data points that we as a company are able to really easily pick up on and help our, our partners actually figure out.
William Tincup: Well, that is that. Thank you.
Wonderful question. A wonderful answer, by the way. Um, Thank Nancy, thank you so much. I know you’re super busy and I love what you’ve built and you’re building and I can’t wait for it to be also be available also, uh, as software, uh, uh, as application is, is, uh, with, with a light services layer, uh, in the future.
So when that happens, please let’s have another show cause I’d love to talk about that. Thanks again for coming on
Nancy Xu: this show. [00:27:00] Absolutely. Thank you so much. And I will say last, um, last tidbit before we, we sign off here we are taking on, um, new partners and we’re constantly looking for, um, fast growing impact driven companies to work with us and we would love to help you hire.
So, um, for anyone who’s listening to the show, if you can email me directly, my email’s, Nancy Moon Hub ai, um, and mention that you heard the show and I’ll personally get you set up with a, with a.
William Tincup: Awesome. Nancy, thank you so much, and thanks for everyone for listening to the show.
Nancy Xu: Thanks so much, Willam. All right.
The Use Case Podcast
Authors
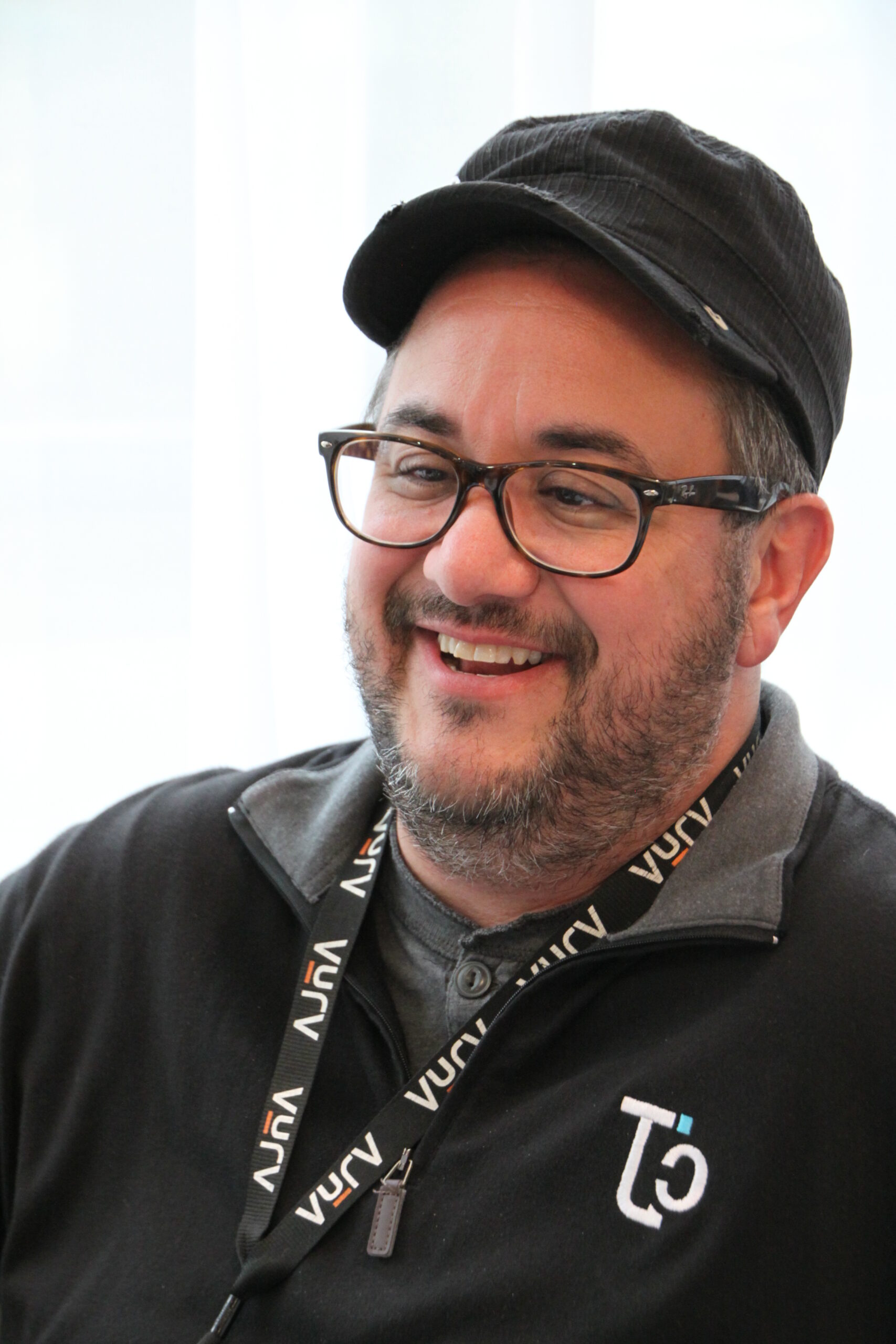
William Tincup
William is the President & Editor-at-Large of RecruitingDaily. At the intersection of HR and technology, he’s a writer, speaker, advisor, consultant, investor, storyteller & teacher. He's been writing about HR and Recruiting related issues for longer than he cares to disclose. William serves on the Board of Advisors / Board of Directors for 20+ HR technology startups. William is a graduate of the University of Alabama at Birmingham with a BA in Art History. He also earned an MA in American Indian Studies from the University of Arizona and an MBA from Case Western Reserve University.
Discussion
Please log in to post comments.
Login