On today’s episode of the RecruitingDaily Podcast, William Tincup speaks to Mathieu and Jen from Snagajob about why AI in hiring may do more harm than good.
Some Conversation Highlights:
Listening time: 26 minutes
Enjoy the podcast?
Thanks for tuning in to this episode of The RecruitingDaily Podcast with William Tincup. Be sure to subscribe through your favorite platform.
Announcer: 00:00 This is Recruiting Daily’s Recruiting Live Podcast, where we look at the strategies behind the world’s best talent acquisition teams. We talk recruiting, sourcing, and talent acquisition. Each week, we take one overcomplicated topic and break it down so that your three year old can understand it. Make sense? Are you ready to take your game to the next level? You’re at the right spot. You’re now entering the mind of a hustler. Here’s your host William Tincup.
William Tincup: 00:34 Ladies and gentlemen is William Tincup, and you’re listening to the Recruiting Daily Podcast. Today, I have Mathieu and Jen on from Snagajob. And our topic today is AI and hiring, why it might do more harm than good. Everyone talks about the positive parts of AI, and there are some positives obviously, but we’ll be talking a little bit maybe about some of the things we’re not thinking about. So why don’t we do some introductions? Mathieu, would you do us a favor and introduce yourself. And Jen, you do the same thing and then introduce Snagajob.
Mathieu Stevens…: 01:06 Yeah, for sure. So for those who don’t know, Snagajob is the largest marketplace for hourly work in the country. So we help about six million workers each month find right fit employment, whether that be full-time, part-time or gig based shift work. And then I am the CEO at Snagajob. I’ve been here just a little under four years.
Jen Clark: 01:29 Hi everybody, I’m Jen. I am the Head of Data Products, and one of my responsibilities is building some of the algorithms that we use to match workers with employers.
William Tincup: 01:42 Awesome. So why don’t we start where we think of the harm. I think with the popular pressure, we probably say biases that we take our own human biases and then we bring them into AI. What do y’all see first? And Jen, we’ll start with you. What do you see as where this could get off the rails pretty quickly in terms of doing more harm than good?
Jen Clark: 02:07 Yeah, I think one of the things that we think a lot about is, it’s really important to frame the problem with anti-bias in mind. And it’s also really important to recognize that bias also exists in the data itself. I think a lot of people don’t really… We often see data as objective, but really it’s a human tool. So making sure that all aspects of AI, however we’re leveraging it, you really take that critical lens to it.
William Tincup: 02:45 That’s fantastic. And Mathieu, do you have any other color commentary?
Mathieu Stevens…: 02:49 Yeah, I’d say, broadly we do believe AI can be a positive good in hiring. It’s just not by no means of cure all. I think the typical pitfalls that you see is more in the approach and application, like a lot of things in life. I think Jen mentioned one, which is, oftentimes firms just don’t have a clear problem statement. What are they actually hoping to achieve? Are they actually trying to increase diversity or are they just replicating existing biases? Second is around not recognizing bias. And what I mean by that is any data that you use in AI involves some form of humans making decisions around either what data to include or what data to exclude. And that process in and of itself leads to unintended bias. And so it’s really important to be auditing those data sets and understanding what bias it’s creating. The third in my mind is around just lack of transparency.
Which is when decisions are made by AI without any sort of explanation, I think it does two things. One, it limits your ability to inspect the process. The other is, I think it reduces the confidence that, at least in hiring either an employer or a worker has. Oftentimes you hear one of the big complaints of AI from employers is, “I just don’t understand how it works. It’s a black box.” And so that lack of transparency, I think is a typical pitfall.
And then the last one, I would say sometimes when people don’t just take into a consideration the human element. And what I mean by that is sometimes a really well intentioned uses and applications of AI say, one way video, which is a pretty common one. But sometimes those things can actually create more anxiety for candidates and users than other approaches.
And so it’s important to balance, Hey, what may be effective versus what’s actually a good consumer experience? I know in our case, we had piloted the use of one way video and what we actually ended up finding is that it was creating a lot of anxiety for workers, more so than more traditional alternatives, whether it be an online questionnaire or a phone based interview. And so even though it had proven effective, it was just as effective as phone based interviews. We actually chose to wind it down. Because we just said, “Hey, it’s not creating the experience that we would want.”
William Tincup: 05:33 There’s like 19 things there to unpack. So we’ll go slow. One of them that I really keyed in on is the rush to AI. And we’ve seen it for years, if we’ve been to any of the HR tech shows, or even just Sherm. You go by someone’s booth and it’s splashed everywhere. And it doesn’t say machine learning or NLP or any of these other things, it’s just AI everything.
And so Jen, I want to get your take on what you see as that rush and why you think that we… Is it just a bright, shiny new object and everyone’s just rushing and they’re not thinking about necessarily the downsides?
Jen Clark: 06:20 Yeah, absolutely. That’s a great question. I’ve actually been in the HR space for most of my career. And I’ve also seen the dynamics that you were talking about, especially at some of the larger conferences. And one of my cautionary tales for a while is, as you mentioned, it can be like a marketing tool, right? It’s a bright, shiny object. We threw some AI in it and it makes people more likely to buy the product or whatever they’re selling. And Mathieu really outlined the things that are really the pitfalls around that type of approach.
I always say, machine learning for machine learning’s sake. If you’re not taking the time to really be thoughtful around the problem that you’re defining and the data that you’re using and how you’re going to explain that to your users really just becomes a marketing tool at that point. And really can have some unintended consequences when as a user, end user of whatever product it is, you don’t exactly know how it’s being used.
William Tincup: 07:30 Right. It’s interesting because I’ve said this for a couple of years, and so I’m not sure the audience, both in HR and in recruiting, if they actually know what AI is, and I’m not sure that the people selling AI actually know what AI is. And I’ve faced a little conflict on this. And I’m like, “Listen, all right.” Even at a conference recently, I said this to somebody and they’re like, “Yeah, you’re not right.” I’m like, “Okay, well,” we’re literally in a hallway, and I said, “Grab somebody and I’m going to put 10 things in front of them. And I just want them to explain the differences between these 10 things.” Bitcoin, blockchain, AI, NLP, this, that, and the other. And they did, they pulled a person in front of me and I said, “Hey, listen, we’re just doing a mall survey. So there’s nothing wrong here.” And we did this bit and the lady just looked at me. She goes, “I have no idea. I have no idea.”
First of all, I could be way off on that. So I get it. But do y’all get that sense from your clients or your customers or prospects that they don’t quite know what AI is?
Mathieu Stevens…: 08:46 I think, listen, AI is just a complicated topic. And so I think a lot of people say, “Hey, I recognize there’s inefficiency in the way that hiring is done today. I believe that technology can play greater role. And I know that AI and other applications or machine learning, subset can have an impact.” And so there unfortunately can be a tendency for sometimes people to misappropriate the use of AI or lean on everything being AI driven. When the reality is there are some applications that are, I think really well suited to machine learning and AI, and there are others where you’d say, “Hey, that may not be the best application of it.”
So I think that’s where I fully get it. I don’t think that I would expect most people to necessarily know the real details of how AI works. Like I said, it’s a pretty difficult thing to understand. I think the onus is on companies, whether they be a Snagajob, whether they be others to better help explain how it’s being used and when it’s being used in really easy to understand terms, and that’s on us.
William Tincup: 10:11 I like that. Jen, that brings me to that question of, do we even talk about the how? Or should we, both as on the vendor side and also for practitioners’ sake, should we even, outside of… And we’ll unpack these in the second in terms of auditing and ethical and transparency, all the issues over there. Should we even get into the how the technology, other than here are the outcomes you should expect?
Jen Clark: 10:38 Yeah. I think to Mathieu’s point, I think we should. And I think the onus is really on the vendors. Is like, I would not expect my customers to be able to talk about the ends and outs of all the different models and the data inputs. But it’s my responsibility and my team’s responsibility to be able to explain thoughtfully and simply how we use those in our product. And I think that is a conversation that is worth pushing on and really bringing out and open and transparent. Rather than, to your point earlier around flashy materials. Really just having an open conversation around what data is being used. In simple terms, what outcomes can be expected from what is being used and where?
William Tincup: 11:37 I love that. So let’s go back, Mathieu, throughout 19 things at once, which is great as a host of a podcast, because now it’s like, “Oh, okay, well here’s the 19 things we should explore.”
Let’s start with auditing from, how often should we for our customers, how often should we be auditing our own internal processes around AI?
Mathieu Stevens…: 12:05 Yeah. I think one of the things that we have committed to, and Jen can talk about a little bit around how we are thinking about approaching probably the trickiest piece, which is auditing for anti-bias for ensuring anti-bias. But we have committed to not just conducting at least an annual audit of our AI models, but additionally, and I think this is an important piece, is actually publishing the results.
William Tincup: 12:32 Oh cool.
Mathieu Stevens…: 12:32 And I think the publishing of the results is important, frankly, to keep us accountable as well as to provide much needed transparency to both workers and employers around, “Hey, this is what we are actually seeing.”
Then Jen, maybe you can talk a little bit about in these situations, how are we thinking about some of the trickier piece, which is, Hey, how do you ensure anti-bias when by nature, you don’t actually want and aren’t collecting demographic data today.
Jen Clark: 13:08 Yeah, absolutely. We’re still working through exactly what this looks like. To Mathieu’s point, this is complex. And to date, we haven’t even collected demographic data because really want to make sure that we’re thinking thoughtfully before we go into that frontier. But some of the ways that we’re thinking about approaching it is really through the same means that we were just talking about. It’s really leveraging community and partnering with select clients to review their own hiring data and having conversations about what that looks like and how do they ensure anti-bias? And how that looks and what do their own audit programs look like?
And then I think what’s really important also internally is you think about a blind study that would be run that’s for a small sample, you get some optional demographic data to calibrate the models again. So basically you say, “Okay, here’s a model, here’s what it predicted. And let’s look at what it did to demographics.” And there’s a bunch of complex techniques that front leading anti-bias, AIethists are looking into. But that’s just simple, tangible ways of just bringing again, the conversation out in the open and saying, “We can’t solve this alone. We have to solve this with our community.”
William Tincup: 14:32 I love that. I love that. So we are talking a little bit and let me ask a different question on the auditing, how frequently should we publish? I love what y’all are doing in terms of both the auditing, but also putting what your findings back in front of the audience to make sure that you’re holding yourself accountable, they can also help you with the accountability as well. How often are y’all doing that? Is that annual report?
Mathieu Stevens…: 15:00 Yeah. Yeah. We believe in annual, which is similar as well to what we do internally, even as it relates to DEI. And so we think the same as it relates to our AI models that we should be doing that.
William Tincup: 15:09 Perfect.
Mathieu Stevens…: 15:09 At least on an annual basis to start.
William Tincup: 15:10 Perfect. Perfect. And then that leads us into transparency. When something in the annual report or the transparency report, when it doesn’t go the way that we think it is. And it just goes sideways and this has happened to everybody. So this is one of those things that we start with the best intentions, all of a sudden we get halfway down the road and it’s like, “Okay, well, no, that didn’t create the… It has an adverse impact, et cetera. Okay. Will we stop doing that?”
Do y’all see that as a part of the reporting process and the transparency process?
Mathieu Stevens…: 15:47 I think it has to be. I think you have to be willing to acknowledge in that where you are seeing bias and then what are the measures that you are taking to mitigate or remove that bias. Again, an example for us is that pilot program that I mentioned that we ran with use of one way video. We said, “Hey, this is where it’s working. This is where it’s not working. We’re now going to wind it down and we’re going to explore two other alternative paths.”
William Tincup: 16:18 Love that. And Jenny, anything to add there on either the auditing or transparency?
Jen Clark: 16:23 Yeah. I think that transparency from an auditing perspective, I think Mathieu’s right. Again, nobody has the answers to this. So being transparent and open about what you’re learning and how you’re trying to actively mitigate is important. I also think that it’s really important to be transparent in your product as well. About showing people your work, like you would a math problem. Where it’s being used actively in the product, what’s being taken into account. And ultimately, I think working towards a place where you’re giving your users the ability to manage their data inputs and how it’s being used. I think because this gets so complex, ultimately the best thing that you can do is sometimes turn over the keys and say, “I want to opt out or I don’t want that piece of information used.” And so I think transparency goes through all levels.
William Tincup: 17:24 I love that. Okay. So you touched on ethical AI and some of the things in ethical AI. How do you all see the future? Maybe not quite right now, but the future of independent audit, having other people look at what you’re doing and then grade you on how you’re doing it and what that looks like? Is that something that you should do internally? Is that something done externally? What’s your take? Not right now, and again, this is a moment in time you might change your mind tomorrow.
Mathieu Stevens…: 17:59 Yeah.
William Tincup: 17:59 What’s your take.
Mathieu Stevens…: 18:00 Jen should weigh in as well. I think the one thing that we have committed to doing is having external expertise. The way we’ve thought about it is less having them do an external audit of the results and more an audit in providing input and guidance on the approach.
That’s the way that we’ve been thinking about it right now. And again, I think the external lens, just like it is in so many other areas, is incredibly helpful to pressure test the team’s thinking.
Jen Clark: 18:34 Yeah. And I would just to add to that, I think Mathieu’s right. Is like you have to have outside eyes to pressure test. But I think it’s also really important from an internal perspective, is that we have, again, there’s a theme here, just open candid conversations with our entire team, not just our leadership to ensure, see something, say something. As like, if something doesn’t seem right in what we’re building or the results that we’re getting in the models, that’s something to flag. So I think that it’s important to have that open conversation from all levels, especially the people that are actively building the product. And for them to be bought in and aware of making sure that we have this goal and we have this end-state in mind and they have to be part of that process.
William Tincup: 19:23 I love that. So questions that practitioners or buyers should ask about AI. Okay. So let’s back up for just a second and go, okay, let’s see it from their perspective. When someone joins this Snagajob family, what should they ask about AI?
Mathieu Stevens…: 19:43 Yeah. Jen, you should weigh in as well. I think what we want to make sure that we are effectively communicating to them… Because again, some people may not know, “Hey, what are the questions I should ask?” Again, I think per earlier, I think the onus is on us and other vendors and partners to be helping folks with this. One is when is it used and how is it being used and what is going into it? And then what are the measures in place to ensure anti-bias and how do I have access to those as they become available?
Jen Clark: 20:27 Yeah. And all of those things I think are really important. I also would add in any kind of vendor partnership, I think it’s good and healthy to ask questions. If we bring up, to Mathieu’s point, if we explain how it’s used or where it’s used, and maybe we use language that is unapproachable, I think that it’s important for us to have a conversation with our customers that, this is exactly what this means in an understandable way. And for our customers to challenge us to constantly be explaining things simply and clearly. Because I think, again, going back to our earlier conversation, it’s very easy to be hand wavy and to provide snake oil when really the onus is on us to be able to explain exactly how it’s being used.
Mathieu Stevens…: 21:24 Yeah, I would just encourage your listeners, a red flag for me, and this is even the case with partners that we talk to in other areas, unrelated to hiring, but just as it relates to running the business. If they can’t easily explain their use of AI and the more they try and complicate the explanation that to me is a bit of a red flag. And so I would just encourage our listeners to pressure test with their partners, “Hey, give me the explanation of how and when you’re using it. So that, in layman’s terms, that I can actually understand.”
And again, from our standpoint, the reason that’s so important is it builds confidence, not just in our partners, but in the day to day users. If you think about the location manager or the HR hiring manager, and they’re getting recommendations that may have been influenced by AI, they need to be able to trust those.
And if they don’t understand it, it’s really difficult to trust it. And again, they don’t need to understand all of the intricacies, they just need to understand the core basics. Like, okay, this is the data that they’re using to do it. This is why it’s being used. I think that’s another oftentimes pitfall with AI is when you utilize data that isn’t easily explainable as to why it’s a criteria. Going back to an example from previous, it’s like, there are a lot of video based solutions that have used in the past facial expressions. We’ve never believed in that as a data element because it’s really difficult to explain, well, why is somebody’s facial expression, a key determinant on how they are on different competency or attitudinal characteristics? It’s just like, even if the data says it’s correlated to things it’s not very easily explainable. And so, that’s one of those kinds of things.
William Tincup: 23:17 And we only have a minute or so left, but we talked to all the harmful, which is great. We should talk about some of the good that y’all see in AI. Right now, even with Snagajob, some of the things you think, okay, this is a low hanging fruit. This is actually really good use for AI. And Jen, we’ll start with you. And then Mathieu, you’ll wrap us up.
Jen Clark: 23:39 Yeah. I get super passionate about this because ultimately we do believe that AI can help both workers and employers find their best fit matches. As we’ve talked about, it’s not a cure all. But we see AI as a partner that can solve for the complexity of the job space, really helping both our workers and our employers navigate all of the inputs that go into finding their best job for someone, interest, availability, preferences, qualifications, while also being a partner to reduce, and hopefully eventually eliminate that bias. That kind of scale, that really is a machine problem and can really help people navigate the complexities.
William Tincup: 24:27 Awesome. Mathieu, anything to add?
Mathieu Stevens…: 24:30 The only thing that I’d add is an example from COVID. And an example of, I think where AI can be incredibly powerful to both. Is if you think about it, there was this incredible displacement of workers in industries. And one of the challenges that many workers had is they might have come from industries, which were basically shut down in COVID. And a lot of them had this immediate reaction of, “Well, I’m not qualified for other roles in different industries.” And where AI can and was incredibly helpful to workers, and then correspondingly to employers, was to basically identify roles that they were well matched for based on their underlying skills and competencies from the positions that they had worked in other industries, that they might not have known about.
And similarly with employers, it was a way to identify candidates who were right fits, who, again, may not have had the historically traditional career in the way that they had looked. They hadn’t come from that industry, but the reality was in what they had done, they had the right set of qualifications. And so I think that’s an example as it relates to career pathing and up-leveling for workers, and over time employers. It’s incredibly powerful, otherwise we saw in COVID, I don’t think would’ve happened.
William Tincup: 25:56 That is fantastic. This whole thing has been fantastic. I think we could have gone on for about another 30 minutes. So Mathieu, thank you for your time. Jen, thank you for your time. And thank you for just your intellect and this is just a wonderful topic.
Mathieu Stevens…: 26:09 Yeah. Thank you so much for having us. So I really, really appreciate it.
Jen Clark: 26:13 Yeah. Thank you so much.
William Tincup: 26:14 Absolutely. Thanks for everyone listening to the Recruiting Daily Podcast. Until next time.
Speaker 1: 26:19 You’ve been listening to the Recruiting Live Podcast by Recruiting Daily. Check out the latest industry podcast, webinars, articles, and news at recruit-
The RecruitingDaily Podcast
Authors
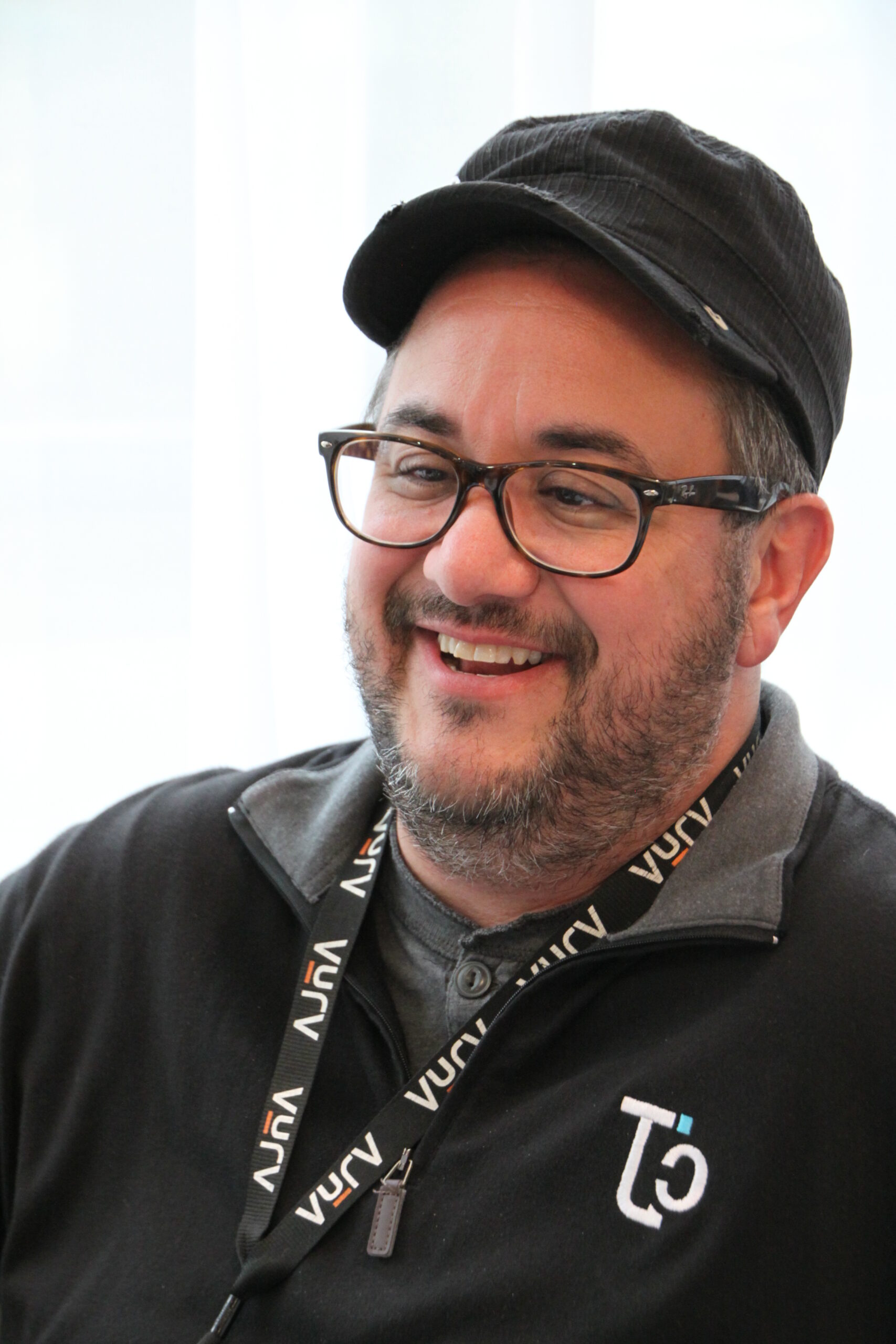
William Tincup
William is the President & Editor-at-Large of RecruitingDaily. At the intersection of HR and technology, he’s a writer, speaker, advisor, consultant, investor, storyteller & teacher. He's been writing about HR and Recruiting related issues for longer than he cares to disclose. William serves on the Board of Advisors / Board of Directors for 20+ HR technology startups. William is a graduate of the University of Alabama at Birmingham with a BA in Art History. He also earned an MA in American Indian Studies from the University of Arizona and an MBA from Case Western Reserve University.
Discussion
Please log in to post comments.
Login