In this episode of the Recruiting Daily Podcast, William Tincup interviews Kiran Snyder, CEO and founder of Textio, about the future of HR tech and its relationship with chat GPT. Textio is a software that helps people write inclusive job posts, recruiting mail, and performance feedback by optimizing their content for inclusion. It goes beyond spelling and grammar and helps users see how other people might receive their writing. On the other hand, chat GPT is an app that uses GPT models to produce conversation-like experiences.
Snyder explains that ChatGPT can conduct interviews and provide automated responses to job applicants but can also be biased towards certain groups of people. Unfortunately, ChatGPT may not be able to handle sensitive conversations. Snyder suggests that HR professionals should use ChatGPT as a tool rather than a solution to their hiring problems.
Kieran Snyder on the future of HR Tech
“We’ve seen people try to write job posts with ChatGPT. It’s really tempting. We’ve seen people try to write sourcing emails. ‘Hey, William, met you at this conference. Got a great role for you,’ That kind of thing. We’ve even seen people try to write higher stakes sort of feedback or performance management content. Everything”
For our most recent podcast episodes: Click Here
Listen & Subscribe on your favorite platform
Apple | Spotify | Google | Amazon
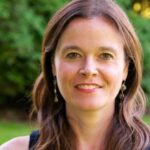
Kieran Snyder
CEO and Co-Founder of Textio. Long-time software product leader, accomplished data writer, recovering academic with a PhD in Linguistics and Cognitive Science from the University of Pennsylvania. Deep experience in product management, product marketing, pricing and licensing, and SaaS across the board, with specific technical strengths in natural language processing and data science.
FollowTextio – ChatGPT and the Future of HR Tech With Kieran Snyder
William Tincup: [00:00:00] This is William Tincup and you’re listening to the Recruiting Daily Podcast. Today we have Kiran on from Textio and we’re, our topic today, which I think is a fascinating topic, is chat, G P T, and the future. Of HR tech, and good God, this thing could be at least a day long, but we’re gonna try and wrap it up in about 25 minutes or so.
And who better than to talk to the founder of Textio? Um, she knows more [00:01:00] about this than, than pretty much anybody I know. So, Karen, would you do us a favor and introduce yourself and Textio?
Kieran Snyder: You bet. So I’m really glad to be here. My name is Kirin Snyder. I use she her pronouns and I am the c e o and one of the two founders of Textio.
I’m so excited about this topic. Uh, at Textio, we have been making software to help people write content about people. At work for a number of years now. So we help our customers write inclusive job posts, recruiting, mail performance feedback, all the nuts and bolts stuff that you’re writing every day at work, and sort of help you optimize it for inclusion, uh, in a way that’s pretty automatic in software.
So, really excited to be here and talk. And I’ve,
William Tincup: I’ve learned, I’ve loved Textio and what y’all have done, uh, for a hundred years now, but for the audience, if they’re not familiar, [00:02:00] um, so you might think of Grammarly, right? Uh, okay. That’s cool for like spelling and grammar. Textio goes, I mean that’s, that’s like table stakes, but it goes much, much, much deeper into the word choices that we make and, uh, and and what other people might view those word choices as.
So I, I remember using Textio a couple years ago and it would, it would tell me, cuz I write you Knowles, man, I write. Right. Shockingly enough, my, my emails, even some of the words that I would use were like, you know, it kind of dialed me back and just made me aware of just word choice, which I thought was, was fantastic.
I know, and you know, back then you, you, you really helped me kind of have a better understanding of my own writing.
Kieran Snyder: Well, thanks you. That is good to hear. And yeah, you, you said it really well. You know, a, a product like Grammarly sort of helps you avoid. [00:03:00] Mortification, you know, helps you get, get, get the coms in the right place, get your grammar in the right place.
Textile really goes a step beyond and helps you see how other people might receive what you’re saying. Right? And that could be about word choice. It could be about structure. It’s really about communication broadly,
William Tincup: and it’s always learning. That’s the, the beauty of it Yes. Is it’s always, it’s always learning and getting better so that as things change, as you change as a writer, it’s always trying to make you better, which, which I love.
I love your work. Thank you, uh, so much for the, what you do with Textio, but let’s talk about chat. G p t, by the way, I hate the name. Um, what, when it first you, you probably saw this, uh, much sooner than probably the, the average, uh, practitioner. What’d you initially think of it and, and how do you, and again, the topic of how do you think that’s gonna influence what we do in hr?
Kieran Snyder: Yeah. Well chat. G [00:04:00] P T is just the front end for a technology that’s been in development for a long time. Right. Uh, and so underneath, I’ll just do a little like terminology check, cuz I think it can be a little confusing for a lot of people who might be listening. So chat, G P T is the app. Uh, where you can go and have some pretty transformative experiences, uh, in conversation.
So you, you go and you type your query into a little box like you’re used to a search and chat, g p t will answer you. Underneath that are the G P T models. Right now it’s G P T three, G P T four is coming soon underneath that, and that’s a large language model and an algorithm that produces everything that you see in chat G P T.
And so as chat G P T was released, you know, it got to a million users faster. We’ve probably all seen the statistics faster. Facebook and Instagram and, and some pretty incredible social media products, [00:05:00] and I think it really opened the eyes of a broader set of people. to the technology that had been in development for a long time.
I think I saw maybe eight weeks after release, 30% of Americans had tried chat g p t at work, which is stunning. , yes. Like that is a, that is a shocking statistic because 30% of Americans are not early technology adopters. So the technologies here, . Um, and I, I do think, I’m excited to dive into what it means for HR Tech specifically, because I’m sure a number of people listening have given it a whirl themselves.
William Tincup: Right? Right. And, and there’s gonna be, like with any technology, there’s gonna be pros and cons, right. Especially emerging technologies. Um, and so let’s just dive right into it. How, how have you, because you got a, a gillion customers, how have you heard from them what, how they’ve used it or. and, and, and also some of the, kind of the cool stories and also some of [00:06:00] the, eh, this didn’t, it didn’t, didn’t work as well as we thought it would with, you know, which, which is fine, you know, experimentation is part of this.
But, so with your, with, with, with yourself, your own company, and even with some of your customers, you don’t have to name ’em of course, but just what have been some of the ways that they’ve.
Kieran Snyder: Well, we are having a lot of conversations about this with our customers. I am spending now a lot of, uh, deep dive time with some of our larger enterprise customers to discuss their strategy here.
So we’ve certainly seen people try to write. Job post with chat g p t. It’s really tempting. Oh God. Oh, you know, we’ve, we’ve seen people try to write sourcing mail, so you know, Hey, William, met you at this conference. Got a great role for you. That kind of thing. Um, we’ve even seen people try to write, uh, higher stakes sort of feedback or performance management content.
And [00:07:00] I actually just. Published a pretty large blog series over the last several weeks. I got one more to go where I went through thousands of examples of each of these with chat G P T to see the patterns of bias that show up. Right, right. Because there’s no doubt, you know, what we’re hearing from customers.
Certainly what we’ve seen ourselves is this. easy and fast. Right? Right. I think the time savings potential for people is really vast. You can, you can get something that is bare bones written extremely quickly. The question is, Can you send it? Can you publish it? Um, right. And are you gonna be happy with the results?
And I think that’s where some of the issues start to
William Tincup: creep in. So it’s, it’s, if we, if we, first of all using it for job post scares the hell out of me. Um, but let’s, let’s say we do it that way. Do you see it as if, if people were to do it, they use it in a fast and and easy way, but it would still need to go [00:08:00] through, cuz it’s not gonna, it’s not gonna correct the bias anytime soon.
right? Because, well, it’s, it’s gotta learn. I mean, even if it were, it’s gotta, it’s gonna be years before it actually learns. What, what biases are there? Right?
Kieran Snyder: Well, it’s really interesting. So if you ask chat g p t to write something generic, like write me a front end engineer job post. Right? What you get out the other side.
It’s not gonna perform well, it’s also not gonna embarrass you. It’s pretty generic, right? Um, you don’t get particular levels of bias, but you also don’t get anything that any real candidate is likely to engage with. as you make these prompts longer and more detailed, you know, you say things like, maybe I’m writing a post for a front end engineer at a healthcare organization in Chicago, right?
Yes. You start adding a little more depth. The level of bias [00:09:00] goes up in direct proportion to the specificity of the prompt, especially around race and. , um, gender bias, uh, is there as well, but race and age bias are the stronger. So the longer the prompt gets right, the more biased the output becomes, which is, you know, kind of the opposite of what you’d wanna see if you’re really using the tool for your day-to-day task.
So you either use it, you get something generic, or you use it with more depth and you get something bias.
William Tincup: So how do you, when you’ve been talking to your enterprise clients, how do you kind of, uh, again, there’s a lot of excitement, a lot of articles, a lot of hype, all that’s all. That’s great. And, uh, but how do you kind of talk and I say talk ’em off the ledge.
It’s not really the right way of phrasing that, but how do you, how do you talk to ’em and say, it is really cool for this not as cool from a bias perspective for these things and. It’s, it’s [00:10:00] easy to get caught up in, in the excitement of any of these, of any tool. I mean, I, I, virtual reality, like it’s easy to get caught up in something and, and just think it’s gonna change the world and not really think about the unintended consequences.
Kieran Snyder: Yeah, I mean, I think the thing that’s most compelling for people is to see the examples. So we talked about job posts, I’ll mention about sourcing mail for a minute. Right? Right. So if, if I ask chat g p t to write me an outreach mail. for, let’s say a machine learning engineer. And I give a set of criteria, you know, where this person spoke, how much experience they have, uh, maybe I say where they live.
Um, and maybe I mentioned that the candidate I’m looking at is black, right? It’s not too hard for chat g p t to write something. And, and by the way, This is truly a subject line that I got in chat. G p T, uh, machine learning engineer wanted bonus points for being black [00:11:00] nearsighted and living in Arizona.
right? .
William Tincup: Oh Lord.
Kieran Snyder: And you know, so certainly when it’s this egregious, most recruiters would not send this , right? They would, they would edit it. The problem is what happens when it’s one click less? Egree. Right. And maybe you don’t know that you’re tokenizing somebody in a way that is problematic. Um, you know, and so what gets written is going to contain issues.
The question is whether you and your team have the tools to spot and fix those issues before you place send and make like a really big mistake. Um, and the degree of tokenization is. very high because the system hasn’t been taught not to do that.
William Tincup: Right, right. Do you, do you see it on the front end of any particular HR technology as, as something that’s done?
Like I was talking to a [00:12:00] gentleman earlier today and he’s built digital workers that basically it’s AI and machine learning, and they go through, Lots of data. So think of like actuary tables, just tons of data, and they find irregular irregularities and then they basically alert a human being to then make sure that it’s irregularity.
And so like, like that’s a wonderful case of saying, okay, you know what? A human being can go, he goes through so much data in an hour or day or whatever, and a machine can go. Much easier. They don’t have to take breaks, you know, et cetera. That’s like a great utility to then see on the front end of something that’s human.
So with with HR Tech, do you see a place for chat, G P T on the front end of something? Uh, again, performance management, you use that as a great, as an example, not a great example. An example. Uh, do you see it in the future? Maybe once. More sophisticated or it’s been used more? [00:13:00] Do you see it on the front end of any of the tools we know?
Kieran Snyder: Well, I know a lot of tools will try to integrate the G P T or other language model technologies into their front end. I mean, that’s definitely happening. One of the, and by the way, I should say like I’m a software girl. I believe in software. I built software because I do think machines are really good at finding patterns that people can’t see.
You know, we’re all the product of our own experiences and that. Biases. The problem with some of these large language models is that they are not designed for the thing you’re writing them for. Right? Right. There’s one language model that is being used to generate job posts. Your letters to Santa Claus.
your performance review, , you know, your product specification, your note to your mom, right? And so there’s no opportunity for a feedback loop that helps you really see how the language you’re choosing is [00:14:00] impacting people in that context. So with job pose, part of, you know, when you’re, if you’re really building sort of a, you talked about learning before, if you’re building a learning, Part of how, you know what’s biased in the job post context is you see which groups of people have actually engaged with these patterns of language before.
If you don’t have that feedback loop, right, you can’t actually detect. Bias in the data set, you won’t know where it is, especially when it’s not egregious. Correct. When it’s subtle stuff. And so the problem with just consuming these large language models off the shelf as is, is they were not built specifically for HR tech, and they certainly weren’t built for specific kinds of writing within HR tech.
And so you will always push. The bias forward. And it’s dangerous because the documents can sound on the surface well-written, professional grammatical. Right, right. And so you might think it’s okay, and in reality, the engagement you [00:15:00] get is likely to be problematic. Well,
William Tincup: just like the, the first time I use TTO for myself, it, it, you know, I, I used it for a job post, uh, a hundred years ago and it, and , it had a lot of color.
Kieran Snyder: Yes, I did ,
William Tincup: I remember, I remember it popping up and I’m like, whoa, wait a minute. And it, and it just, it taught me again, it kind of, it, it, I didn’t know, uh, it is not like I was doing anything on purpose. I just didn’t know. And so with these large language models, it’s not going to be able to get, inform you, the, the reader.
It’s not gonna be able to inform the person that’s getting it. And so it’s not gonna get smart. in that way. Uh, of, of
Kieran Snyder: learning those things. That’s right. And, and as biases get pointed out, you know, there’s kind of a famous example that’s been written about a lot on the internet in the way the different ways that chat, G p t writes about Donald Trump and Joe Biden.
Right. A lot of [00:16:00] controversy. , um, open AI is trying to address bias problems. Right. But the way they do it is by adding individual rules. Each time a case is pointed out, it’s a little bit like having a toothache and taking Advil every day instead of just going to get the root canal. Right? Right. They, they sort of stick on band-aid style individual rules.
And so when you write anything, That it hasn’t anticipated, and people have endless creative capacity. Oh yeah. For bias. Oh, yes. Oh. The bias comes through. So actually I wrote, um, an article, uh, asking chat g p t to write workplace Valentine’s. Right? And which, you know, scenarios that hasn’t been considered before.
And it turns out when you write Valentine’s towards. Men or male coworkers, you get words like drive and ambition. Yep. And strength and professional. And when you write Valentine’s towards female employees, you get [00:17:00] grace, determination, smile. Yeah. Lovely. Yeah. And so when Jet Chachi PT, or or the language model has an added rules specifically to filter these out, all the stereotypes and the underlying dataset come through right?
In anything you’re.
William Tincup: I, what’s, what’s, again in those words, you don’t know those words or triggers, uh, for people, uh, unless you’re informed. So, uh, it’s it’s, I I think you’re right. I know you’re right. Actually, there’s gonna be an, an onslaught of folks in, in our industry that are gonna try to use it in some way with large lingual models.
Either, you know, chat G P T or OpenAI or, or whatever else. They’re gonna try to use it. , but. It’s thinking about what are the out, what are the potential negative outcomes? And again, both of us are fans, uh, but not, maybe not fans for its use in work. Uh, well, or
Kieran Snyder: [00:18:00] I think, I think you can use, I think there are ways to incorporate the technology, but you can’t do it without marrying the technology.
Right. With the domain specific knowledge and data. If you just take it as is. If you’re an HR tech vendor, your customers will write all kinds of problematic and undermining things. It will undermine their chances of success if you can partner. the core technology with a better data set, right? , you have the chance to do something really interesting.
You know, we, we didn’t talk about performance feedback, but one of the interesting cases that when I was looking at this, are the gender assumptions that come through. So if I ask chat g p t to say, write feedback for a bubbly receptionist, guess what? Gender it assumes that person is. And it replies, right?
Yeah, just bubbly, very consistent. Like some of these really push through and so if you just take it as is, um, you end [00:19:00] up with a lot of
William Tincup: problems, right? I think that’s, and and I, we’re gonna see that as true as it gets placed anywhere else in the organization, outplacement or succession or compensa, anywhere that it gets placed, that people think of that creatively again, it’s gonna be, it’s gotta have another layer, which is great.
That makes it more refined and more, again, less bias, uh, or exactly. or we’re going to, we’re going to, we’re gonna find ourselves cuz biases. I think this is what, you’re the expert, but I’ve always thought of biases as not finite. , uh, more that we’ve learned more about biases as we go along. Like, you know, uh, I’ve written about biases, like, kind of dumb biases, but like tattoo bias or music bias or things like that.
Yeah. But it’s like, as we learn these things, it’s not, it’s not like it’s, they learn and you’re done. You’re just, you’re, you’re just, it’s like an appealing an onion if, if, if you’ve ever done that. It’s just, it keeps exposing more biases, [00:20:00] uh, and that, that we can learn.
Kieran Snyder: That’s exactly right. You know, and, and said people are really, I think you’re right.
It’s not finite and people are really creative . Right. You know, when I, when I asked Chap g p t to write a job post for an HR business partner who’s a regular church goer, it happily took the prompts. Right When in real life, unless you’re hiring for somebody who’s working at a church, that’s probably not a relevant characteristic.
Right. And to con, you know, to consider. And so, but it’s because there’s been no specific role-based programming against that bias. And so it happily, you know, chirps along and gives you something that pulls that all the way through the job post, which of course right, you would not wanna publish.
William Tincup: And in large language models, they’re not gonna fix that on the front.
That’s not their, that’s not what they’re trying to precisely. Like if you precisely put churchgoer, uh, it, it’s, it’s actually illegal to talk about that in, in most cases, uh, to ask people what religion they are, et cetera. So if, if, [00:21:00] if done cor, if, if, if those large language models we’re trying to eradicate Bris, when you put in churchgoer, he would then flag.
And, and that’s right. Right. But it’s, that’s right. That’s not what they’re, that’s not their, that’s not what they’re trying to fix. That’s not, that’s not, um, that’s not their. Well,
Kieran Snyder: and especially not in the core data set, they said they, they can add individual rules when enough people on the internet point out a problem.
Right. Um, you know, if, if we point out that bubbly receptionists are always assumed to be female, then they can add a rule to make sure that that particular assumption’s not baked in. But what about an organized kindergarten? . What if you didn’t think about that one? Yeah. Or a strong construction worker.
What if you didn’t think about that one? Right? There’s just so many combinations where the underlying data has bias baked. So,
William Tincup: so the last question for, and this, this is really for practitioners to just consider is using it and trying it, uh, in [00:22:00] their personal lives. Uh, maybe using it and trying it in their professional lives, but really making sure that anything that goes out to candidates or employees has been vetted like more than you would in something normal.
Uh, What other advice would you give practitioners as they, as they play with both kind of the, we’ll just say large language models, cuz I, I think that’s mm-hmm. a better way to think of these things than just one brand. Um, how do, how do you want them to kind of listen to this and go, okay, hey listen, the, the sky’s not following, it’s just there’s new technology.
With new technology, there’s going to be things that we like. There’s gonna be things that are great. There’s also gonna be things that, you know, Unintended or intended, uh, either way that you need to kind of be careful about. So what would you, when you’re, again, talking to your prospects and your customers, what advice do you have with these large language models?
Kieran Snyder: So the place that I [00:23:00] feel, um, they have a lot of use and applicability is in. Um, really accelerating the writing of things that are fairly boilerplate, where you have very detailed notes. So if you have a bunch of notes about your parental leave policy, for example, and you need to turn your notes into a well-written document.
this technology’s gonna really help you accelerate it. You still need to read it and edit it, right, right before it gets published. But if you’re writing things where you’ve got detailed notes, the content’s fairly, you know, uh, documentation oriented, I think this can be a big accelerator for teams. And I think, you know, it’s great to explore it if you are writing sensitive content, you know, to a candidate, to an employee.
Certainly if you’re talking about somebody’s career. , all the same biases that you’re worried your team has in the first place are only amplified here. Because remember, the training data is just [00:24:00] drawn from what real people have already written, and some of those people are your coworkers, right? It’s just the, it’s just the way it works.
And so you can’t, um, Sort of set it and forget it pr, you know, produce it and publish it without a all the same mechanisms and techniques that you’re already using to address bias in the organization. The extra level of risk with this comes in that people are not actually writing it themselves. So they’re not going through the internal interrogation process as they’re writing to think, do I really wanna say it this?
Um, you know, you skip that whole opportunity for the people themselves to be learning as they’re putting their words on paper. And so it does require an extra level of scrutiny before you get it out the door.
William Tincup: I’m pro, I’ll probably go further, um, because even in that example where you have a lot of notes around the parental leave, uh, thing, and you’re building either a policy or a part of the handbook, et cetera.
even that language [00:25:00] can be, even, even that language that gets published can be littered with biases for sure. So I, I’m probably gonna put a two, three year kind of window on this to just say, yes, you can create it. You’re gonna then spend time with the document and make sure that it doesn’t have those things.
So
Kieran Snyder: it’s, yeah. Well I don’t even think that’s two or three years I think. That’s an indefinite thing. Like Yeah, you good point. The, the same way, like you wouldn’t put your name on something and send it out the door without making sure it’s good today. Like this, this doesn’t alleviate that responsibility.
You, you know, if anything, it increases the responsibility because you had less direct oversight over what was getting written yourself.
William Tincup: It’s, it’s what we learn about, uh, contracts. You don’t sign a contract unless you read it. That’s right. There you go. , same, same bit, same scenario. Karen, this has been wonderful.
I love Textio. I love what you’ve built and I love your knowledge about this in particular, just carving out time for [00:26:00] us. Thank you so much. You’re
Kieran Snyder: welcome.
William Tincup: Lots of fun. Absolutely, and thanks for everyone listening. We’ll see you next, Tom.
The RecruitingDaily Podcast
Authors
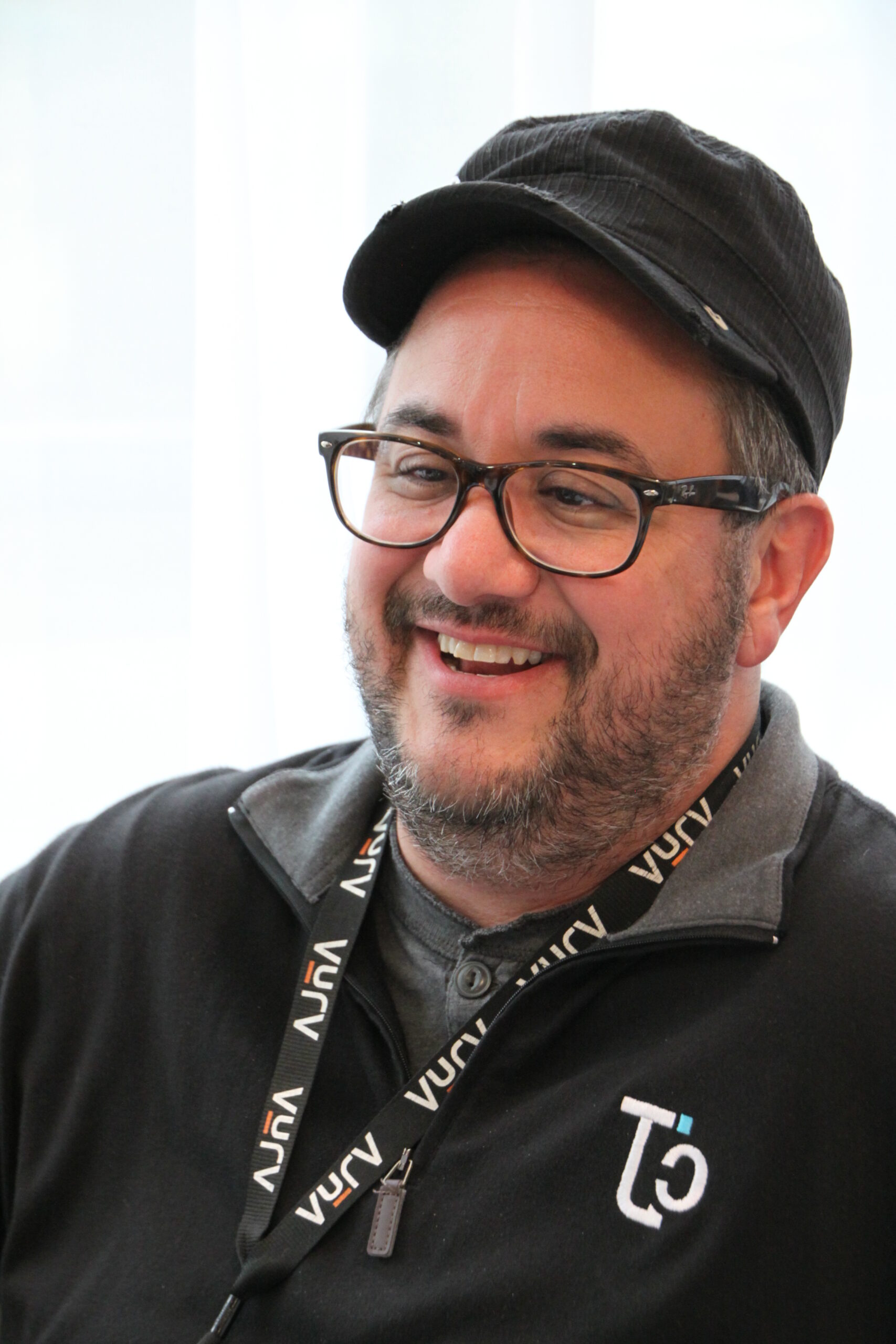
William Tincup
William is the President & Editor-at-Large of RecruitingDaily. At the intersection of HR and technology, he’s a writer, speaker, advisor, consultant, investor, storyteller & teacher. He's been writing about HR and Recruiting related issues for longer than he cares to disclose. William serves on the Board of Advisors / Board of Directors for 20+ HR technology startups. William is a graduate of the University of Alabama at Birmingham with a BA in Art History. He also earned an MA in American Indian Studies from the University of Arizona and an MBA from Case Western Reserve University.
Discussion
Please log in to post comments.
Login